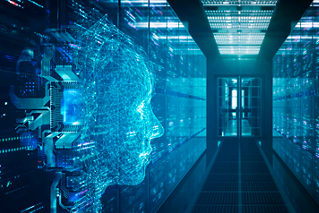
Making the Case for Software Development with AI
Tue, 12 Sep 2023 00:50:39 -0000
|Read Time: 0 minutes
There are an astounding number of use cases for artificial intelligence (AI), across nearly every industry and spanning outcomes that range from productivity to security to user experience and more. Many of these use cases are discussed in a Dell white paper on Generative AI in the Enterprise, a collaboration with NVIDIA that enables high performance, scalable, and modular architectures for generative AI solutions.
Some of the most impactful use cases to date are in the field of software development. Here, AI can be used for tasks such as automating code development, detecting issues, correcting erroneous code, and assisting coders. AI can provide suggestions and automated code completion.
One remarkable solution at the forefront of this AI-driven transformation is Codeium Enterprise, a high-quality, enterprise-grade, and exceptionally performant AI-powered code acceleration toolkit. A unique aspect of Codeium Enterprise is that it can be deployed entirely on-premises using Dell hardware. This solution offers enterprises a competitive advantage through faster development in their software teams, with minimal setup and maintenance requirements.
Codeium Enterprise Essentials
Codeium Enterprise is designed to address the key challenges faced by businesses aiming to enhance worker productivity in software development. It leverages industry-leading generative AI capabilities but is accessible to developers without requiring pre-existing AI expertise.
Codeium can be used with existing codebases or for generating new code and offers several essential capabilities including:
- Personalized Coding Assistance: Codeium Enterprise is a personalized AI-powered assistant tailored to enterprise software development teams.
- Industry-Leading Generative AI: It leverages industry-leading generative AI capabilities to enhance developer productivity across the entire software development life cycle.
- Ease of Use: Codeium Enterprise is accessible to developers without requiring pre-existing AI expertise. It can be used with existing codebases or for generating new code.
Codeium Enterprise builds upon the base Codeium Individual product, used by hundreds of thousands of developers. Codeium Individual provides features like autocomplete, chat, and search to assist developers throughout the software development process. The toolkit seamlessly integrates into more than 40 Integrated Development Environments (IDEs) for over 70 programming languages.
Codeium Enterprise on Dell Infrastructure
Collaborating with Dell Technologies, Codeium offers powerful yet affordable hardware configurations that are satisfactory for running Codeium Enterprise on-premises. This approach ensures that both intellectual property and data remain secure within the enterprise's environment.
Dell Technologies can power Codeium Enterprise with PowerEdge servers of various GPU configurations, depending on your development teams’ sizes. Larger development teams can use multiple servers since Codeium is a horizontally scalable system and supports multinode deployments.
Dell Experience
During the initial deployment to software developers within Dell, the results were overwhelmingly positive. After two brief weeks of use following the initial rollout, developers were polled and reported the following feedback:
- 78% of developers reported creating the first revision of code more quickly.
- 89% reported decreased context switching and improved flow state (“being in the zone”).
- 92% reported improved productivity overall.
- 100% wanted to continue using the toolset.
Did we say that the results were overwhelmingly positive?
Conclusion
In a rapidly evolving technological landscape, generative AI holds the potential to revolutionize software development. Codeium Enterprise, running on Dell infrastructure, provides a comprehensive solution designed to meet the requirements of enterprises. It can enhance developer productivity, ensure data and IP security, adhere to licensing compliance, offers transparency through analytics, and minimizes costs. Codeium Enterprise is a great choice for enterprises seeking to leverage generative AI for productivity while maintaining control and security in their software development.
Incorporating Codeium Enterprise into your software development processes is not just a competitive advantage; it is a strategic move towards staying at the forefront of innovation in the software industry.
For more information, view the joint Solution Brief or contact the Codeium team.
Related Blog Posts
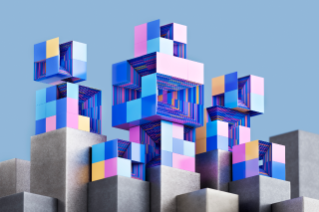
Dell Validated Design Guides for Inferencing and for Model Customization – March ’24 Updates
Fri, 15 Mar 2024 20:16:59 -0000
|Read Time: 0 minutes
Continuous Innovation with Dell Validated Designs for Generative AI with NVIDIA
Since Dell Technologies and NVIDIA introduced what was then known as Project Helix less than a year ago, so much has changed. The rate of growth and adoption of generative AI has been faster than probably any technology in human history.
From the onset, Dell and NVIDIA set out to deliver a modular and scalable architecture that supports all aspects of the generative AI life cycle in a secure, on-premises environment. This architecture is anchored by high-performance Dell server, storage, and networking hardware and by NVIDIA acceleration and networking hardware and AI software.
Since that introduction, the Dell Validated Designs for Generative AI have flourished, and have been continuously updated to add more server, storage, and GPU options, to serve a range of customers from those just getting started to high-end production operations.
A modular, scalable architecture optimized for AI
This journey was launched with the release of the Generative AI in the Enterprise white paper.
This design guide laid the foundation for a series of comprehensive resources aimed at integrating AI into on-premises enterprise settings, focusing on scalable and modular production infrastructure in collaboration with NVIDIA.
Dell, known for its expertise not only in high-performance infrastructure but also in curating full-stack validated designs, collaborated with NVIDIA to engineer holistic generative AI solutions that blend advanced hardware and software technologies. The dynamic nature of AI presents a challenge in keeping pace with rapid advancements, where today's cutting-edge models might become obsolete quickly. Dell distinguishes itself by offering essential insights and recommendations for specific applications, easing the journey through the fast-evolving AI landscape.
The cornerstone of the joint architecture is modularity, offering a flexible design that caters to a multitude of use cases, sectors, and computational requirements. A truly modular AI infrastructure is designed to be adaptable and future-proof, with components that can be mixed and matched based on specific project requirements and which can span from model training, to model customization including various fine-tuning methodologies, to inferencing where we put the models to work.
The following figure shows a high-level view of the overall architecture, including the primary hardware components and the software stack:
Figure 1: Common high-level architecture
Generative AI Inferencing
Following the introductory white paper, the first validated design guide released was for Generative AI Inferencing, in July 2023, anchored by the innovative concepts introduced earlier.
The complexity of assembling an AI infrastructure, often involving an intricate mix of open-source and proprietary components, can be formidable. Dell Technologies addresses this complexity by providing fully validated solutions where every element is meticulously tested, ensuring functionality and optimization for deployment. This validation gives users the confidence to proceed, knowing their AI infrastructure rests on a robust and well-founded base.
Key Takeaways
- In October 2023, the guide received its first update, broadening its scope with added validation and configuration details for Dell PowerEdge XE8640 and XE9680 servers. This update also introduced support for NVIDIA Base Command Manager Essentials and NVIDIA AI Enterprise 4.0, marking a significant enhancement to the guide's breadth and depth.
- The guide's evolution continues into March 2024 with its third iteration, which includes support for the PowerEdge R760xa servers equipped with NVIDIA L40S GPUs.
- The design now supports several options for NVIDIA GPU acceleration components across the multiple Dell server options. In this design, we showcase three Dell PowerEdge servers with several GPU options tailored for generative AI purposes:
- PowerEdge R760xa server, supporting up to four NVIDIA H100 GPUs or four NVIDIA L40S GPUs
- PowerEdge XE8640 server, supporting up to four NVIDIA H100 GPUs
- PowerEdge XE9680 server, supporting up to eight NVIDIA H100 GPUs
The choice of server and GPU combination is often a balance of performance, cost, and availability considerations, depending on the size and complexity of the workload.
- This latest edition also saw the removal of NVIDIA FasterTransformer, replaced by TensorRT-LLM, reflecting Dell’s commitment to keeping the guide abreast of the latest and most efficient technologies. When it comes to optimizing large language models, TensorRT-LLM is the key. It ensures that models not only deliver high performance but also maintain efficiency in various applications.
The library includes optimized kernels, pre- and postprocessing steps, and multi-GPU/multi-node communication primitives. These features are specifically designed to enhance performance on NVIDIA GPUs.
It uses tensor parallelism for efficient inference across multiple GPUs and servers, without the need for developer intervention or model changes.
- Additionally, this update includes revisions to the models used for validation, ensuring users have access to the most current and relevant information for their AI deployments. The Dell Validated Design guide covers Llama 2 and now Mistral as the foundation models for inferencing with this infrastructure design with Triton Inference Server:
- Llama 2 7B, 13B, and 70B
- Mistral
- Falcon 180B
- Finally (and most importantly) performance test results and sizing considerations showcase the effectiveness of this updated architecture in handling large language models (LLMs) for various inference tasks. Key takeaways include:
- Optimized Latency and Throughput—The design achieved impressive latency metrics, crucial for real-time applications like chatbots, and high tokens per second, indicating efficient processing for offline tasks.
- Model Parallelism Impact—The performance of LLMs varied with adjustments in tensor and pipeline parallelism, highlighting the importance of optimal parallelism settings for maximizing inference efficiency.
- Scalability with Different GPU Configurations—Tests across various NVIDIA GPUs, including L40S and H100 models, demonstrated the design’s scalability and its ability to cater to diverse computational needs.
- Comprehensive Model Support—The guide includes performance data for multiple models (as we already discussed) across different configurations, showcasing the design’s versatility in handling various LLMs.
- Sizing Guidelines—Based on performance metrics, updated sizing examples are available to help users determine the appropriate infrastructure based on their specific inference requirements (these guidelines very welcome)
All this highlights Dell’s commitments and capability to deliver high-performance, scalable, and efficient generative AI inferencing solutions tailored to enterprise needs.
Generative AI Model Customization
The validated design guide for Generative AI Model Customization was first released in October 2023, anchored by the PowerEdge XE9680 server. This guide detailed numerous model customization methods, including the specifics of prompt engineering, supervised fine-tuning, and parameter-efficient fine-tuning.
The updates to the Dell Validated Design Guide from October 2023 to March 2024 included the initial release, the addition of validated scenarios for multi-node SFT and Kubernetes in November 2023, updated performance test results, and new support for PowerEdge R760xa servers, PowerEdge XE8640 servers, and PowerScale F710 all-flash storage as of March 2024.
Key Takeaways
- The validation aimed to test the reliability, performance, scalability, and interoperability of a system using model customization in the NeMo framework, specifically focusing on incorporating domain-specific knowledge into Large Language Models (LLMs).
- The process involved testing foundational models of sizes 7B, 13B, and 70B from the Llama 2 series. Various model customization techniques were employed, including:
- Prompt engineering
- Supervised Fine-Tuning (SFT)
- P-Tuning, and
- Low-Rank Adaptation of Large Language Models (LoRA)
- The design now supports several options for NVIDIA GPU acceleration components across the multiple Dell server options. In this design, we showcase three Dell PowerEdge servers with several GPU options tailored for generative AI purposes:
- PowerEdge R760xa server, supporting up to four NVIDIA H100 GPUs or four NVIDIA L40S GPUs. While the L40S is cost-effective for small to medium workloads, the H100 is typically used for larger-scale tasks, including SFT.
- PowerEdge XE8640 server, supporting up to four NVIDIA H100 GPUs.
- PowerEdge XE9680 server, supporting up to eight NVIDIA H100 GPUs.
As always, the choice of server and GPU combination depends on the size and complexity of the workload.
- The validation used both Slurm and Kubernetes clusters for computational resources and involved two datasets: the Dolly dataset from Databricks, covering various behavioral categories, and the Alpaca dataset from OpenAI, consisting of 52,000 instruction-following records. Training was conducted for a minimum of 50 steps, with the goal being to validate the system's capabilities rather than achieving model convergence, to provide insights relevant to potential customer needs.
The validation results along with our analysis can be found in the Performance Characterization section of the design guide.
What’s Next?
Looking ahead, you can expect even more innovation at a rapid pace with expansions to the Dell’s leading-edge generative AI product and solutions portfolio.
For more information, see the following resources:
- Dell Generative AI Solutions
- Dell Technical Info Hub for AI
- Generative AI in the Enterprise white paper
- Generative AI Inferencing in the Enterprise Inferencing design guide
- Generative AI in the Enterprise Model Customization design guide
- Dell Professional Services for Generative AI
~~~~~~~~~~~~~~~~~~~~~
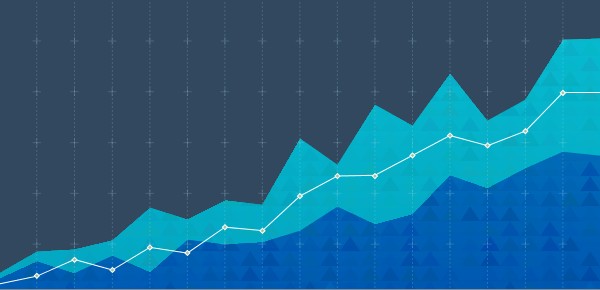
Dell Technologies Shines in MLPerf™ Stable Diffusion Results
Tue, 12 Dec 2023 14:51:21 -0000
|Read Time: 0 minutes
Abstract
The recent release of MLPerf Training v3.1 results includes the newly launched Stable Diffusion benchmark. At the time of publication, Dell Technologies leads the OEM market in this performance benchmark for training a Generative AI foundation model, especially for the Stable Diffusion model. With the Dell PowerEdge XE9680 server submission, Dell Technologies is differentiated as the only vendor with a Stable Diffusion score for an eight-way system. The time to converge by using eight NVIDIA H100 Tensor Core GPUs is 46.7 minutes.
Overview
Generative AI workload deployment is growing at an unprecedented rate. Key reasons include increased productivity and the increasing convergence of multimodal input. Creating content has become easier and is becoming more plausible across various industries. Generative AI has enabled many enterprise use cases, and it continues to expand by exploring more frontiers. This growth can be attributed to higher resolution text to image, text-to-video generations, and other modality generations. For these impressive AI tasks, the need for compute is even more expansive. Some of the more popular generative AI workloads include chatbot, video generation, music generation, 3D assets generation, and so on.
Stable Diffusion is a deep learning text-to-image model that accepts input text and generates a corresponding image. The output is credible and appears to be realistic. Occasionally, it can be hard to tell if the image is computer generated. Consideration of this workload is important because of the rapid expansion of use cases such as eCommerce, marketing, graphics design, simulation, video generation, applied fashion, web design, and so on.
Because these workloads demand intensive compute to train, the measurement of system performance during their use is essential. As an AI systems benchmark, MLPerf has emerged as a standard way to compare different submitters that include OEMs, accelerator vendors, and others in a like-to-like way.
MLPerf recently introduced the Stable Diffusion benchmark for v3.1 MLPerf Training. It measures the time to converge a Stable Diffusion workload to reach the expected quality targets. The benchmark uses the Stable Diffusion v2 model trained on the LAION-400M-filtered dataset. The original LAION 400M dataset has 400 million image and text pairs. A subset of those images (approximately 6.5 million) is used for training in the benchmark. The validation dataset is a subset of 30 K COCO 2014 images. Expected quality targets are FID <= 90 and CLIP>=0.15.
The following figure shows a latent diffusion model[1]:
Figure 1: Latent diffusion model
[1] Source: https://arxiv.org/pdf/2112.10752.pdf
Stable Diffusion v2 is a latent diffusion model that combines an autoencoder with a diffusion model that is trained in the latent space of the autoencoder. MLPerf Stable Diffusion focuses on the U-Net denoising network, which has approximately 865 M parameters. There are some deviations from the v2 model. However, these adjustments are minor and encourage more submitters to make submissions with compute constraints.
The submission uses the NVIDIA NeMo framework, included with NVIDIA AI Enterprise, for secure, supported, and stable production AI. It is a framework to build, customize, and deploy generative AI models. It includes training and inferencing frameworks, guard railing toolkits, data curation tools, and pretrained models, offering enterprises an easy, cost effective, and a fast way to adopt generative AI.
Performance of the Dell PowerEdge XE9680 server and other NVIDIA-based GPUs on Stable Diffusion
The following figure shows the performance of NVIDIA H100 Tensor Core GPU-based systems on the Stable Diffusion benchmark. It includes submissions from Dell Technologies and NVIDIA that use different numbers of NVIDIA H100 GPUs. The results shown vary from eight GPUs (Dell submission) to 1024 GPUs (NVIDIA submission). The following figure shows the expected performance of this workload and demonstrates that strong scaling is achievable with less scaling loss.
Figure 2: MLPerf Training Stable Diffusion scaling results on NVIDIA H100 GPUs from Dell Technologies and NVIDIA
End users can use state-of-the-art compute to derive faster time to value.
Conclusion
The key takeaways include:
- The latest released MLPerf Training v3.1 measures Generative AI workloads like Stable Diffusion.
- Dell Technologies is the only OEM vendor to have made an MLPerf-compliant Stable Diffusion submission.
- The Dell PowerEdge XE9680 server is an excellent choice to derive value from Image Generation AI workloads for marketing, art, gaming, and so on. The benchmark results are outstanding for Stable Diffusion v2.
MLCommons Results
https://mlcommons.org/benchmarks/training/
The preceding graphs are MLCommons results for MLPerf IDs 3.1-2019, 3.1-2050, 3.1-2055, and 3.1-2060.
The MLPerf™ name and logo are trademarks of MLCommons Association in the United States and other countries. All rights reserved. Unauthorized use strictly prohibited. See www.mlcommons.org for more information.