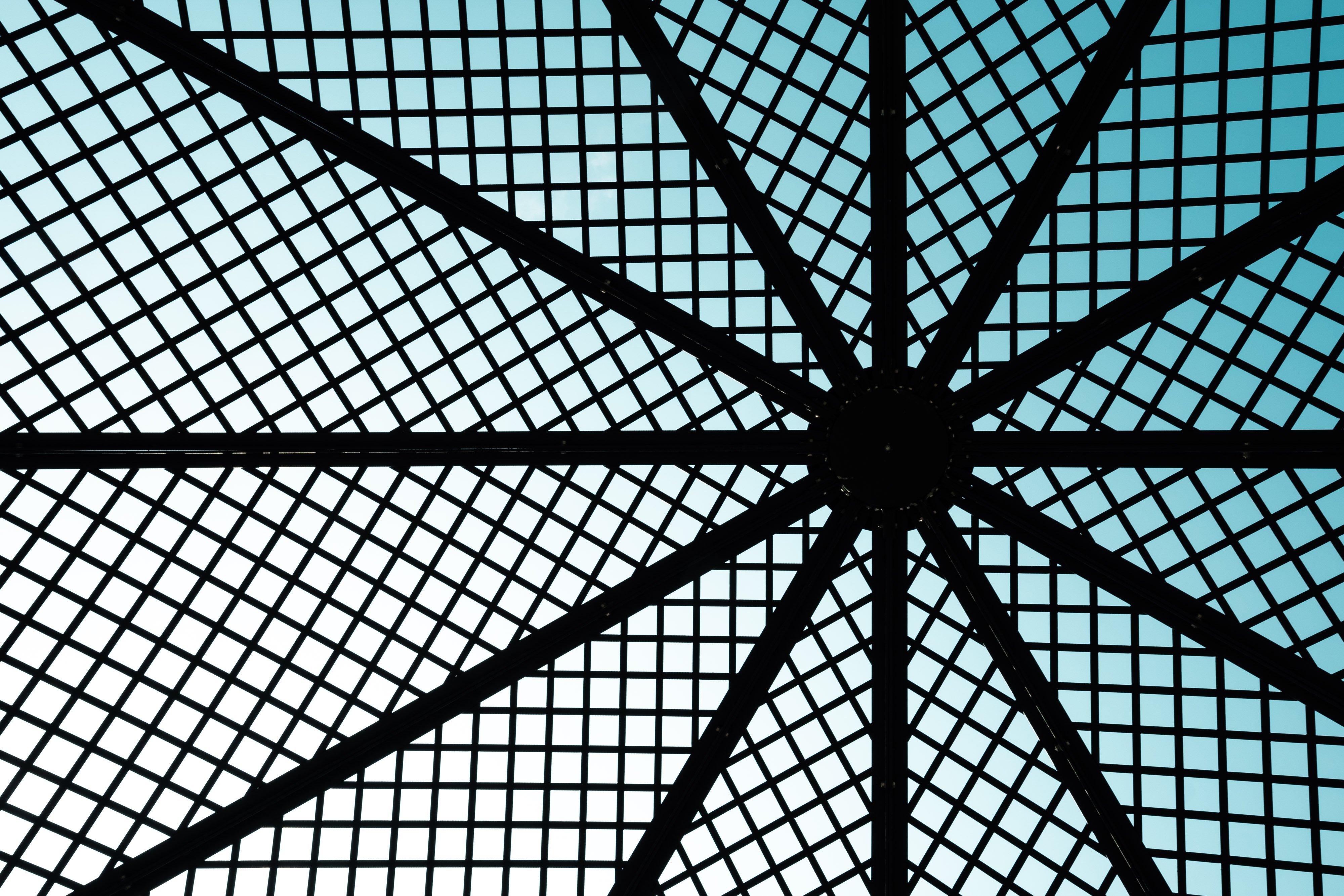
Virtualized GPU Instances on Dell EMC PowerEdge Platforms for Compute Intensive Workloads
Download PDFMon, 16 Jan 2023 13:44:27 -0000
|Read Time: 0 minutes
Summary
In this DfD we address a common problem that is faced by IT teams across different organizations – being able to efficiently share and utilize NVIDIA GPU resources across different teams and projects.
AI adoption is growing in many organizations leading to increased demand of GPU accelerated compute instances. We explore how IT teams can leverage existing investment in virtualized infrastructure combined with NVIDIA Virtual GPU software to provide optimized and secure GPU-ready compute environments for AI researcher and engineers.
Motivation for GPU Virtualization
The requirement and demand for GPU accelerated compute instances is steadily rising in all organizations, driven primarily by rise of AI and Deep Learning (DL) techniques to realize increased efficiencies and improve customer interactions. IT environments continue to adopt virtualization to run all workloads and address requirements of providing secure and agile compute capabilities to end users. NVIDIA Virtual GPU software (previously referred to as GRID) enables virtualizing a physical GPU and allows it to be shared across multiple virtual machines. The rising demand for GPU accelerated compute instances can be achieved by virtualizing GPUs and deploying cost effective GPU accelerated VM instances. Enabling a centralized and hosted solution in the data center provides the security and scalability that is critical to enterprise customers.
Figure 1. GPU enabled VM instances using GPU Pass-Though and GPU Virtualization (vGPU)
NVIDIA Virtual GPU software enables virtual GPUs to be created on a Dell EMC server with NVIDIA GPUs that can be shared across multiple virtual machines. Better utilization and sharing are achieved by transforming a one-to-one relationship from GPU to user to one- to-many.
Traditionally, the IT best practices for compute-intensive (non-graphical) VM instances leveraged GPU pass-through shown in the left half of Figure 1. In a VMware environment, this is referred to as the VM DirectPath I/O mode of operation. It allows the GPU device to be accessed directly by the guest operating system, bypassing the ESXi hypervisor. This provides a level of performance of a GPU on vSphere that is very close to its performance on a native system (within 4-5%).
The main reasons for using the passthrough approach to expose GPUs on vSphere are:
- Simplicity: It is straightforward to allocate GPUs to a VM using pass-though and offer GPU acceleration benefits to end users
- Dedicated use: there is no need for sharing the GPU among different VMs, because a single application will consume one or more full GPUs
- Replicate public cloud instances: public cloud instances use GPU pass-through, and end user wants the same environment in an on-premises datacenter
- A single virtual machine can make use of multiple physical GPUs in passthrough mode
An important point to note is that the passthrough option for GPUs works without third-party software driver being loaded into the ESXi hypervisor.
Disadvantages of GPU passthrough is as follows:
- The entire GPU is dedicated to that VM and there is no sharing of GPUs amongst the VMs on a server.
- Advanced vSphere features of vMotion, Distributed Resource Scheduling (DRS) and Snapshots are not allowed with this form of using GPUs with a virtual machine.
Overview of NVIDIA vGPU Platform
GPU virtualization (NVIDIA vGPU) addresses limitations of pass-through but was traditionally deployed to accelerate virtualized profession graphics applications, virtual desktop instances or remote desktop solutions. NVIDIA added support for AI, DL and high-performance computing (HPC) workloads in GRID 9.0 that was released in summer 2019. It also changed vGPU licensing to make it more amenable for compute use cases. GRID vPC/vApps and Quadro vDWS are licensed by concurrent user, either as a perpetual license or yearly subscription. Since vComputeServer is for server compute workloads, the license is tied to the GPU rather than a user and is therefore licensed per GPU as a yearly subscription. For more information about NVIDIA GRID software, see http://www.nvidia.com/grid.
Figure 2 shows the different components of the Virtual GPU software stack.
Figure 2. GPU enabled VM instances using GPU Pass- Though and GPU Virtualization (vGPU)
NVIDIA GPU Virtualization software transforms a physical GPU installed on a server to create virtual GPUs (vGPU) that can be shared across multiple virtual machines. The focus in this paper is on the use of GPUs for compute workloads using vComputeServer profile introduced in GRID 9. We are not looking at GPU usage for professional graphics or virtual desktop infrastructure (VDI) that will leverage Quadro vDWS or GRID vPC and vAPP profiles. GRID vPC/vApps and Quadro vDWS are client compute products for virtual graphics designed for knowledge workers and professional graphics use. vComputeServer is targeted for compute-intensive server workloads, such as AI, deep learning, and Data Science.
In an ESXi environment, the lower layers of the stack include the NVIDIA Virtual GPU Manager, that is loaded as a VMware Installation Bundle (VIB) into the vSphere ESXi hypervisor. An additional guest OS NVIDIA vGPU driver is installed within the guest operating system of your virtual machine.
Using the NVIDIA vGPU technology with vSphere provides options during creation of the VMs to dedicate a full GPU device(s) to one virtual machine or to allow partial sharing of a GPU device by more than one virtual machine.
IT admins will pick between the options depending on the application and user requirements:
- Partial GPUs: For AI dev environments a data scientist VM will not need the power of full GPU
- GPU sharing: IT admins want GPUs to be share by more than one team of users simultaneously
- High priority applications: dedicate a full GPU or multiple GPUs to one VM
The different editions of the vGPU driver are described next.
NVIDIA virtual GPU Software is available in four editions that deliver accelerated virtual desktops to support the needs of different workloads.
IT administrators can configure VMs using vComputeServer (vCS) profiles to deploy GPU compute instances on top of Dell EMC PowerEdge servers configured with NVIDIA V100 or T4 GPUs. Details of vCS GPU profile and list of Dell EMC Servers that can be used to run VMs accelerated using vCS GPU profiles is provided in the following tables. IT teams have a range of options in terms of vGPU profiles, GPU Models and supported Dell platforms to accommodate the compute requirements of their customer workloads.
vComputeServer Features and Deployment Patterns
vComputeServer was designed to complement existing GPU virtualization capabilities for graphics and VDI and address the needs of the data centers to virtualize compute-intensive workloads such as AI, DL and HPC. As part of addressing the needs of compute-intensive workloads, vCS introduced GPU aggregation inside a VM (multi vGPU support in a VM), GPU P2P support for NVLink, container support using NGC and support for application, VM, and host-level monitoring. A few of the key features are:
Management and monitoring: Admins can use the VMware management tools like VMware vSphere to manage GPU servers, with visibility at the host, VM and app level. GPU-enabled virtual machines can be migrated with minimal disruption or downtime.
Multi vGPU support: Administrators can now combine management benefits of vGPU and leverage the compute capability of scaling-out jobs across multiple GPUs by leveraging multi vGPU support in vComputeServer. Multiple vGPUs can now be deployed in a single virtual machine to scale application performance and speed up production workflows.
Support for NGC Software: vComputeServer supports NVIDIA NGC GPU-optimized software for deep learning, machine learning, and HPC. NGC software includes containers for the popular AI and data science software, validated and optimized by NVIDIA, as well as fully-tested containers for HPC applications and data analytics. NGC also offers pre-trained models for a variety of common AI tasks that are optimized for NVIDIA Tensor Core GPUs. This allows data scientists, developers, and researchers to reduce deployment times focus on building solutions, gathering insights, and delivering business value.
Deploying Virtualized GPU Instances for Compute Intensive Workloads
In this paper we covered the benefits of deploying virtualized VMs that can leverage GPU compute for accelerating emerging workloads like AI, Deep Learning and HPC. Customers that care about highest performance can leverage virtualized instances of NVIDIA V100 GPU in their VMs and also aggregate multiple vGPUs on Dell PE-C4140 server to get increased performance using GPU aggregation capability of vComputeServer profile. Customers concerned about cost can share a GPU between multiple users by leveraging smaller vGPU profiles (upto 16 vGPU profiles can be created from a single V100 or T4 GPU).
Related Documents
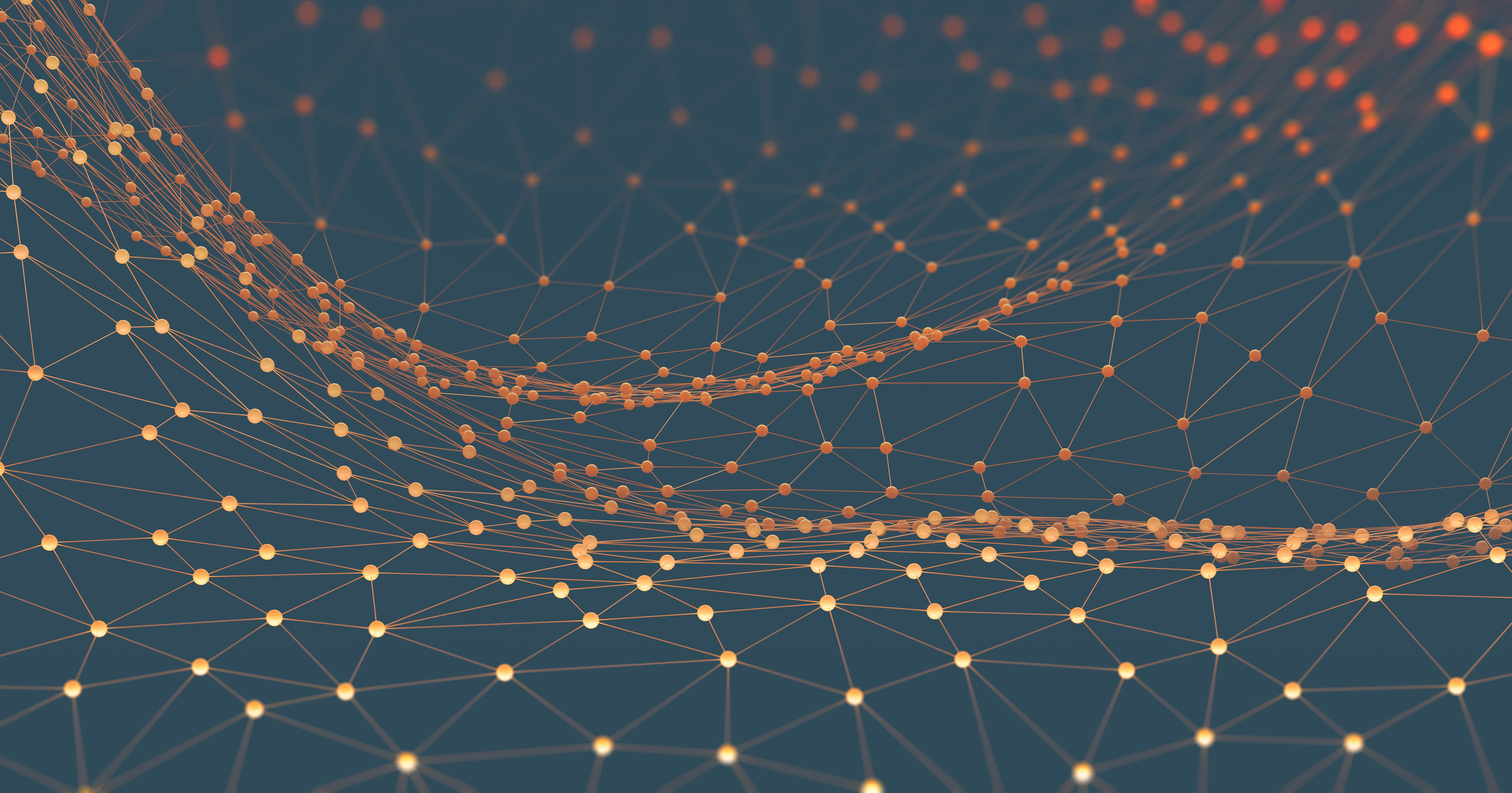
The Latest GPUs of 2022
Mon, 16 Jan 2023 13:44:30 -0000
|Read Time: 0 minutes
And How We Recommend Applying Them to Enable Breakthrough Performance
Summary
Dell Technologies offers a wide range of GPUs to address different workloads and use cases. Deciding on which GPU model and PowerEdge server to purchase, based on intended workloads, can become quite complex for customers looking to use GPU capabilities. It is important that our customers understand why specific GPUs and PowerEdge servers will work best to accelerate their intended workloads. This DfD informs customers of the latest and greatest GPU offerings in 2022, as well as which PowerEdge servers and workloads we recommend to enable breakthrough performance.
PowerEdge servers support various GPU brands and models. Each model is designed to accelerate specific demanding applications by acting as a powerful assistant to the CPU. For this reason, it is vital to understand which GPUs on PowerEdge servers will best enable breakthrough performance for varying workloads. This paper describes the latest GPUs as of Q1 2022, shown below in Figure 1, to help educate PowerEdge customers on which GPU is best suited for their specific needs.
GPU Model | Number of Cores | Peak Double Precision (FP64) | Peak Single Precision (FP32) | Peak Half Precision (FP16) | Memory Size / Bus | Memory Bandwidth | Power Consumption |
A2 | 2560 | N/A | 4.5 TFLOPS | 18 TFLOPS | 16GB GDDR6 | 200 GB/s | 40-60W |
A16 | 1280 x4 | N/A | 4.5 TFLOPS x4 | 17.9 TFLOPS x4 | 16GB GDDR6 x4 | 200 GB/s x4 | 250W |
A30 | 3804 | 5.2 TFLOPS | 10.3 TFLOPS | 165 TFLOPS | 24GB HBM2 | 933 GB/s | 165W |
A40 | 10752 | N/A | 37.4 TFLOPS | 149.7 TFLOPS | 48GB GDDR6 | 696 GB/s | 300W |
MI100 | 7680 | 11.5 TFLOPS | 23.1 TFLOPS | 184.6 TFLOPS | 32GB HBM2 | 1.2 TB/s | 300W |
A100 PCIe | 6912 | 9.7 TFLOPS | 19.5 TFLOPS | 312 TFLOPS | 80GB HBM2e | 1.93 TB/s | 300W |
A100 SXM2 | 6912 | 9.7 TFLOPS | 19.5 TFLOPS | 312 TFLOPS | 40GB HBM2 | 1.55 TB/s | 400W |
A100 SXM2 | 6912 | 9.7 TFLOPS | 19.5 TFLOPS | 312 TFLOPS | 80GB HBM2e | 2.04 TB/s | 500W |
T4 | 2560 | N/A | 8.1 TFLOPS | 65 TFLOPS | 16GB GDDR6 | 300 GB/s | 70W |
Figure 1 – Table comparing 2022 GPU specifications
NVIDIA A2
The NVIDIA A2 is an entry-level GPU intended to boost performance for AI-enabled applications. What makes this product unique is its extremely low power limit (40W-60W), compact size, and affordable price. These attributes position the A2 as the perfect “starter” GPU for users seeking performance improvements on their servers. To benefit from the performance inferencing and entry-level specifications of the A2, we suggest attaching it to mainstream PowerEdge servers, such as the R750 and R7515, which can host up to 4x and 3x A2 GPUs respectively. Edge and space/power constrained environments, such as the XR11, are also recommended, which can host up to 2x A2 GPUs. Customers can expect more PowerEdge support by H2 2022, including the PowerEdge R650, T550, R750xa, and XR12.
Supported Workloads: AI Inference, Edge, VDI, General Purpose Recommended Workloads: AI Inference, Edge, VDI Recommended PowerEdge Servers: R750, R7515, XR11
NVIDIA A16
The NVIDIA A16 is a full height, full length (FHFL) GPU card that has four GPUs connected together on a single board through a Mellanox PCIe switch. The A16 is targeted at customers requiring high-user density for VDI environments, because it shares incoming requests across four GPUs instead of just one. This will both increase the total user count and reduce queue times per request. All four GPUs have a high memory capacity (16GB DDR6 for each GPU) and memory bandwidth (200GB/s for each GPU) to support a large volume of users and varying workload types. Lastly, the NVIDIA A16 has a large number of video encoders and decoders for the best user experience in a VDI environment.
To take full advantage of the A16s capabilities, we suggest attaching it to newer PowerEdge servers that support PCIe Gen4. For Intel-based PowerEdge servers, we recommend the R750 and R750xa, which support 2x and 4x A16 GPUs, respectively. For AMD-based PowerEdge servers, we recommend the R7515 and R7525, which support 1x and 3x A16 GPUs, respectively.
Supported Workloads: VDI, Video Encoding, Video Analytics Recommended Workloads: VDI Recommended PowerEdge Servers: R750, R750xa, R7515, R7525
NVIDIA A30
The NVIDIA A30 is a mainstream GPU offering targeted at enterprise customers who seek increased performance, scalability, and flexibility in the data center. This powerhouse accelerator is a versatile GPU solution because it has excellent performance specifications for a broad spectrum of math precisions, including INT4, INT8, FP16, FP32, and FP64 models. Having the ability to run third- generation tensor core and the Multi-Instance GPU (MIG) features in unison further secures quality performance gains for big and small workloads. Lastly, it has an unconventionally low power budget of only 165W, making it a viable GPU for virtually any PowerEdge server.
Given that the A30 GPU was built to be a versatile solution for most workloads and servers, it balances both the performance and pricing to bring optimized value to our PowerEdge servers. The PowerEdge R750, R750xa, R7525, and R7515 are all great mainstream servers for enterprise customers looking to scale. For those requiring a GPU-dense server, the PowerEdge DSS8440 can hold up to 10x A30s and will be supported in Q1 2022. Lastly, the PowerEdge XR12 can support up to 2x A30s for Edge environments.
Supported Workloads: AI Inference, AI Training, HPC, Video Analytics, General Purpose Recommended Workloads: AI Inference, AI Training Recommended PowerEdge Servers: R750, R750xa, R7525, R7515, DSS8440, XR12
NVIDIA A40
The NVIDIA A40 is a FHFL GPU offering that combines advanced professional graphics with HPC and AI acceleration to boost the performance of graphics and visualization workloads, such as batch rendering, multi-display, and 3D display. By providing support for ray tracing, advanced shading, and other powerful simulation features, this GPU is a unique solution targeted at customers that require powerful virtual and physical displays. Furthermore, with 48GB of GDDR6 memory, 10,752 CUDA cores, and PCIe Gen4 support, the A40 will ensure that massive datasets and graphics workload requests are moving quickly.
To accommodate the A40s hefty power budget of 300W, we suggest customers attach it to a PowerEdge server with ample power to spare, such as the DSS8440. However, if the DSS8440 is not possible, the PowerEdge R750xa, R750, R7525, and XR12 are also compatible with the A40 GPU and will function adequately so long as they are using PSUs with adequate power output. Lastly, populating A40 GPUs within the PowerEdge T550 is also a great play for customers who want to address visually demanding workloads outside the traditional data center.
Supported Workloads: Graphics, Batch Rendering, Multi-Display, 3D Display, VR, Virtual Workstations, AI Training, AI Inference Recommended Workloads: Graphics, Bach Rendering, Multi-Display Recommended PowerEdge Servers: DSS8440, R750xa, R750, R7525, XR12, T550
NVIDIA A100
The NVIDIA A100 focuses on accelerating HPC and AI workloads. It introduces double-precision tensor cores that significantly reduce HPC simulation run times. Furthermore, the A100 includes Multi-Instance GPU (MIG) virtualization and GPU partitioning capabilities, which benefit cloud users looking to use their GPUs for AI inference and data analytics. The newly supported sparsity feature can also double the throughput of tensor core operations by exploiting the fine- grained structure in DL networks. Lastly, A100 GPUs can be inter-connected either by NVLink bridge on platforms like the R750xa and DSS8440, or by SXM4 on platforms like the PowerEdge XE8545, which increases the GPU-to- GPU bandwidth when compared to the PCIe host interface.
The PowerEdge DSS8440 is a great server for the A100, as it provides ample power and can hold the most GPUs. If not the DSS8440, we would suggest using the PowerEdge XE8545, R750xa, or R7525. Please note that only the 80GB model is supported for PCIe connections, and be sure to provide plenty of power to accommodate the A100s 300W/400W power requirements.
Supported Workloads: HPC, AI Training, AI Inference, Data Analytics, General Purpose Recommended Workloads: HPC, AI Training, AI Inference, Data Analytics Recommended PowerEdge Servers: DSS8440, XE8545, R750xa, R7525
AMD MI100
The AMD MI100 value proposition is similar to the A100 in that it will best accelerate HPC and AI workloads. At 11.5 TFLOPS, its FP64 performance is industry-leading for the acceleration of HPC workloads. Similarly, at 23.1 TFLOPs, the FP32 specifications are more than sufficient for any AI workload. Furthermore, the MI100 supports 32GB of high-bandwidth memory (HBM2) to enable a whopping 1.2TB/s of memory bandwidth. In a nutshell, this GPU is designed to tackle complex, data-intensive HPC and AI workloads for enterprise customers.
The AMD MI100 is qualified on both the Intel-based PowerEdge R750xa, which supports up to 4x MI100 GPUs, and the AMD- based PowerEdge R7525, which supports up to 3x MI100 GPUs. We highly recommend adopting a powerful PSU for either server, as the MI100 also has a massive power consumption of 300W.
Supported Workloads: HPC, AI Training, AI Inference, ML/DL Recommended Workloads: HPC, AI Training, AI Inference Recommended PowerEdge Servers: R750xa, R7525
Conclusion
The GPUs we are recommending in this list offer a wide variety of features that are designed to accelerate a diverse range of server workloads. A PowerEdge server configured with the most appropriate GPU will enable intended customer workloads to use these features in concert with other system components to yield the best performance. We hope this discussion of the latest 2022 GPUs, as well as our recommendations for Dell PowerEdge servers and workloads, will help customers choose the most appropriate GPU for their data center needs and business goals.
Learn More
Dell PowerEdge Accelerated Servers and Accelerators Dell eBook
Demystifying Deep Learning Infrastructure Choices using MLPerf Benchmark Suite HPC at Dell
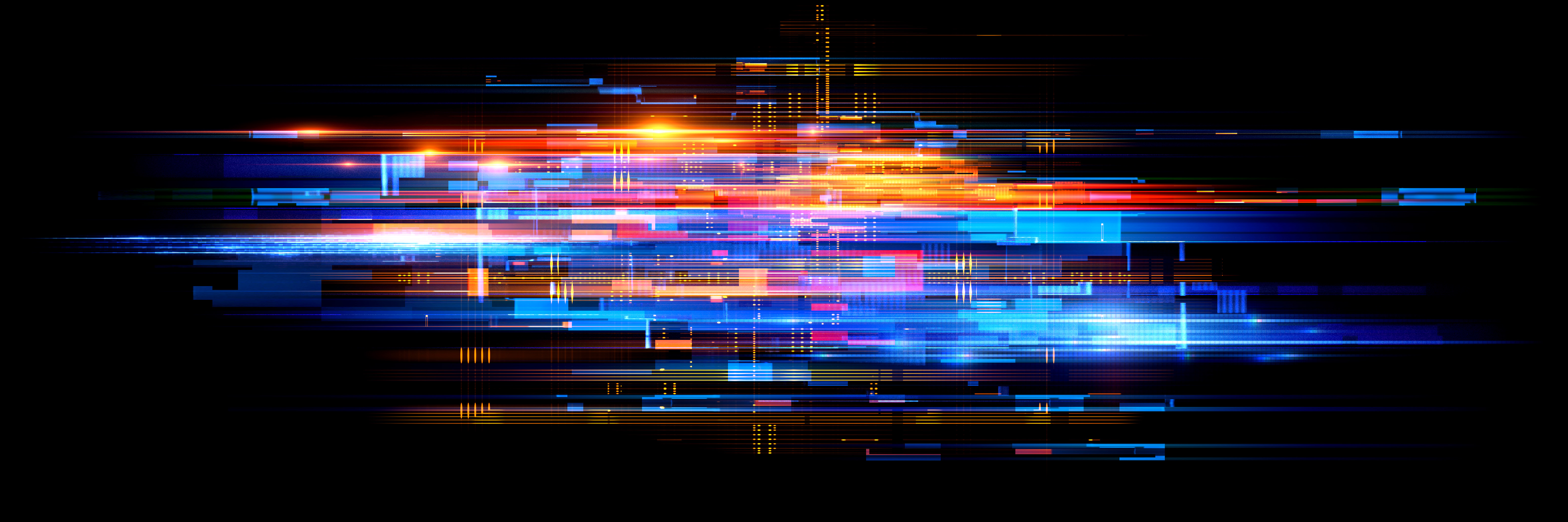
Achieving Significant Virtualization Performance Gains with New 16G Dell® PowerEdge™ R760 Servers
Thu, 25 Jan 2024 17:43:01 -0000
|Read Time: 0 minutes
Summary
With the latest Dell PowerEdge R760 16G servers utilizing the PCIe® 5.0 interface to connect networking and storage to the CPU, there are great performance increases in data movement over previous PCIe generations. These improvements can be utilized by hyperconverged infrastructures running on these servers.
This Direct from Development (DfD) tech note presents a generational server performance comparison in a virtualized environment comparing new 16G Dell PowerEdge R760 servers deployed with new KIOXIA CM7 Series SSDs with prior generation 14G Dell PowerEdge R740xd servers deployed with prior generation KIOXIA CM6 Series SSDs.
As presented by the test results, the latest Dell generation PowerEdge servers perform the same amount of work in less time and deliver faster performance in a virtualized environment when compared with prior PCIe server generations.
Market positioning
Data center infrastructures typically fall into three categories: traditional, converged and hyperconverged. Hyperconverged infrastructures enable users to add compute, memory and storage requirements as needed, delivering the flexibility of horizontal and vertical scaling. However, many virtual machine (VM) configurations run in converged infrastructures, and their ability to scale is often difficult when VM clusters require more storage.
VMware®, Inc. enables hyperconverged infrastructures through VMware ESXi™ and VMware vSAN™ platforms. The VMware ESXi platform is a popular enterprise-grade virtualization platform that scales compute and memory as needed and provides simple management of large VM clusters. The VMware vSAN platform enables the infrastructure to transition from converged to hyperconverged, delivering incredibly fast performance since storage is local to the servers themselves. The platforms support a new VMware vSAN Express Storage Architecture™ (ESA) that has gone through a series of optimizations to utilize NVMe™ SSDs more efficiently than in the past.
Product features
Dell PowerEdge 760 Rack Server (Figure 1)
Specifications: https://www.delltechnologies.com/asset/en-us/products/servers/technical-support/poweredge-r760-spec-sheet.pdf.
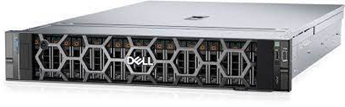
Figure 1: Side angle of Dell PowerEdge 760 Rack Server1
KIOXIA CM7 Series Enterprise NVMe SSD (Figure 2) Specifications:https://americas.kioxia.com/en-us/business/ssd/enterprise-ssd.html.
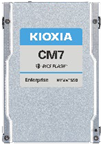
Figure 2: Front view of KIOXIA CM7 Series SSD2
PCIe 5.0 and NVMe 2.0 specification compliant; Two configurations: CM7-R Series (read intensive), 1 Drive Write Per Day3 (DWPD), up to 30,720 gigabyte4 (GB) capacities and CM7-V Series (higher endurance mixed use), 3 DWPD, up to 12,800 GB capacities.
Performance specifications: SeqRead = up to 14,000 MB/s; SeqWrite = up to 7,000 MB/s; RanRead = up to 2.7M IOPS; RanWrite = up to 600K IOPS.
Hardware/Software test configuration
The hardware and software equipment used in this virtualization comparison (Figure 3):
Server Information | ||
Server Model | Dell PowerEdge R7605 | Dell PowerEdge R740xd6 |
No. of Servers | 3 | 3 |
BIOS Version | 1.3.2 | 2.18.1 |
CPU Information | ||
CPU Model | Intel® Xeon® Gold 6430 | Intel Xeon Silver 4214 |
No. of Sockets | 2 | 2 |
No. of Cores | 64 | 24 |
Frequency (in gigahertz) | 2.1 GHz | 2.2 GHz |
Memory Information | ||
Memory Type | DDR5 | DDR4 |
Memory Speed (in megatransfers per second) | 4,400 MT/s | 2,400 MT/s |
Memory Size (in gigabytes) | 16 GB | 32 GB |
No. of DIMMs | 16 | 12 |
Total Memory (in gigabytes) | 256 GB | 384 GB |
SSD Information | ||
SSD Model | KIOXIA CM7-R Series | KIOXIA CM6-R Series |
Form Factor | 2.5-inch7 | 2.5-inch |
Interface | PCIe 5.0 x4 | PCIe 4.0 x4 |
No. of SSDs | 12 | 12 |
SSD Capacity (in terabytes4) | 3.84 TB | 3.84 TB |
Drive Write(s) Per Day (DWPD) | 1 | 1 |
Active Power | 25 watts | 19 watts |
Operating System Information | ||
Operating System (OS) | VMware ESXi | VMware ESXi |
OS Version | 8.0.1, 21813344 | 8.0.1, 21495797 |
VMware vCenter® Version | 8.0.1.00200 | 8.0.1.00200 |
Storage Type | vSAN ESA | vSAN ESA |
Load Generator Information (Test Software) | ||
Load Generator | HyperConverged Infrastructure Benchmark (HCIBench) | HCIBench |
Load Generator Version | 2.8.2 | 2.8.2 |
Figure 3: Hardware/Software configuration used in the comparison
Set-up and test procedures
Set-up:
The latest VMware ESXi 8.0 operating system was installed on all hosts.
Two clusters were created in VMware’s vCenter management interface with ‘High Availability’ and ‘Distributed Resource Scheduler’ disabled for testing.
Each Dell PowerEdge R760 host was added into a cluster - then each Dell PowerEdge R740xd host was added into a separate cluster.
VMkernel adapters were set up to have VMware vMotion™ migration, provisioning, management and the VMware vSAN platform enabled for both test configurations.
In the VMware vSAN configurations, twelve KIOXIA CM7 Series drives were added for the Dell PowerEdge R760 cluster (four drives per server), and twelve KIOXIA CM6 Series drives were added for the Dell PowerEdge R740xd cluster (four drives per server). The default storage policy was set to ‘vSAN ESA Default Policy – RAID 5’ for both configurations.
The HCIBench load generator (virtual appliance) was then imported and configured on the network.
Test procedures:
The latest VMware ESXi 8.0 operating system was installed on all hosts.
Six tests were run on each cluster – four performance tests and two power consumption tests as follows:
Performance tests:
IOPS: This metric measured the number of Input/Output operations per second that the system completed. Throughput: This metric measured the amount of data transferred per second to and from the storage devices.
Read Latency: This metric measured the time it took to perform a read operation. It included the average time it took for the load generator to not only issue the read operation, but also the time it took to complete the operation and receive a ‘successfully completed’ acknowledgement.
Write Latency: This metric measured the time it took to perform a write operation. It included the average time it took for the load generator to not only issue the write operation, but also the time it took to complete the operation and receive a ‘successfully completed’ acknowledgement.
Power consumption tests:
IOPS per Watt: This metric measured the amount of IOPS performed in conjunction with the power consumed by the cluster.
Throughput per Watt: This metric measured the amount of throughput performed in conjunction with the power consumed by the cluster.
For the four performance tests, the following five workloads were run with the test results recorded. For the two power consumption tests, the latter four workloads were run with the test results recorded.
100% Sequential Write (256K block size, 1 thread): This workload is representative of a data logging use case. 100% Random Read (4K block size, 4 threads): This workload is representative of a read cache system.
Random 70% Read / 30% Write (4K block size, 4 threads): This workload is representative of a common mixed read/write ratio used in commercial database systems.
Random 50% Read /50% Write (4K block size, 4 threads): This workload is representative of other common IT use cases such as email.
Blender (block sizes/threads vary): This workload is representative of a mix of many types of sequential and random workloads at various block sizes and thread counts as VMs request storage against the vSAN storage pool.
Test results8
IOPS (Figure 4): The results are in IOPS - the higher result for each is better.
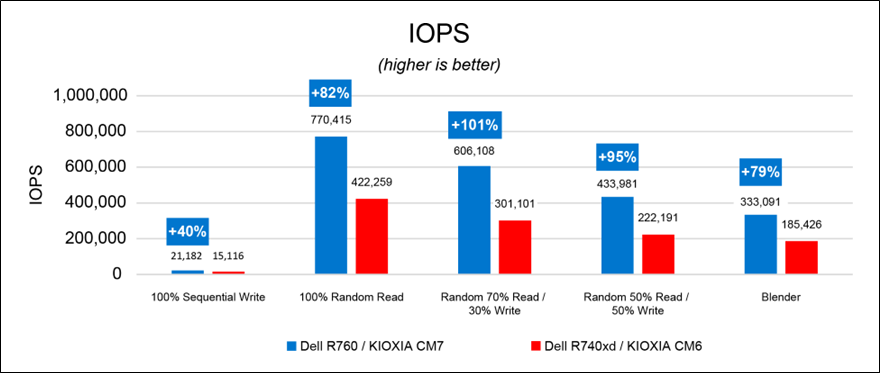
Figure 4: IOPS results
Throughput (Figure 5): The results are in megabytes per second (MB/s) - the higher result for each is better.
Figure 5: throughput results
Read Latency (Figure 6): The results are in milliseconds (ms) - the lower result for each is better. The 100% sequential write workloads for both configurations were not included for this test as the workload does not include read operations.
Figure 6: read latency results
Write Latency (Figure 7): The results are in milliseconds - the lower result for each is better. The 100% random read workloads for both PCIe configurations were not included for this test as the workload does not include write operations.
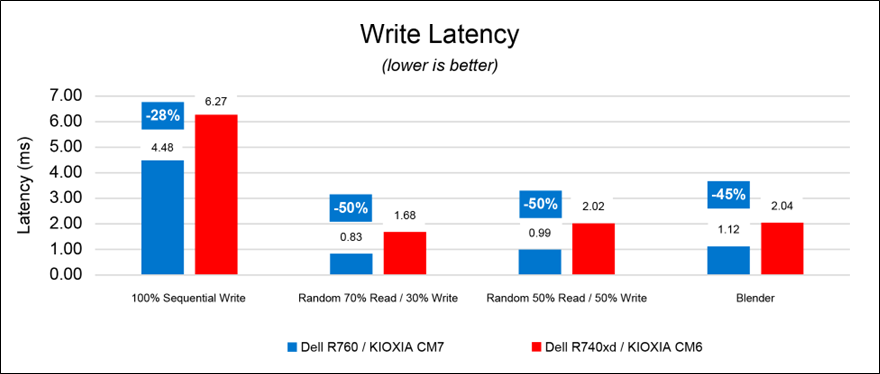
Figure 7: write latency results
IOPS per Watt (Figure 8): The results show the amount of IOPS performed per power consumed by the cluster and are in IOPS per watt (IOPS/W). The higher result for each is better.
Figure 8: IOPS per watt results
Throughput per Watt (Figure 9): The results show the amount of throughput performed per power consumed by the cluster and are in MB/s per watt (MBps/W). The higher result for each is better.
Figure 9: throughput per watt results
Final analysis
The Dell PowerEdge R760 servers equipped with new KIOXIA CM7 Series enterprise NVMe SSDs outperformed the Dell PowerEdge 740xd servers and SSDs in IOPS, throughput and latency. They also delivered higher performance per watt. With the newer generation of Dell PowerEdge servers, there are notable performance increases associated with hyperconverged infrastructures that directly affect server, CPU, memory and storage performance when compared with prior generations.
References
Footnotes
1. The product image shown is a representation of the design model and not an accurate product depiction.
2. The product image shown was provided with permission from KIOXIA America, Inc. and is a representation of the design model and not an accurate product depiction.
3. Drive Write Per Day (DWPD) means the drive can be written and re-written to full capacity once a day, every day for five years, the stated product warranty period. Actual results may vary due to system configuration, usage and other factors. Read and write speed may vary depending on the host device, read and write conditions and file size.
4. Definition of capacity - KIOXIA Corporation defines a megabyte (MB) as 1,000,000 bytes, a gigabyte (GB) as 1,000,000,000 bytes and a terabyte (TB) as 1,000,000,000,000 bytes. A computer operating system, however, reports storage capacity using powers of 2 for the definition of 1Gbit = 230 bits = 1,073,741,824 bits, 1GB = 230 bytes = 1,073,741,824 bytes and 1TB = 240 bytes = 1,099,511,627,776 bytes and therefore shows less storage capacity. Available storage capacity (including examples of various media files) will vary based on file size, formatting, settings, software and operating system, and/or pre-installed software applications, or media content. Actual formatted capacity may vary.
5. The Dell PowerEdge R760 server features a PCIe 4.0 backplane.
6. The Dell PowerEdge R740xd server features a PCIe 3.0 backplane.
7. 2.5-inch indicates the form factor of the SSD and not its physical size.
8. Read and write speed may vary depending on the host device, read and write conditions and file size.
Trademarks
Dell and PowerEdge are registered trademarks or trademarks of Dell Inc.
Intel and Xeon are registered trademarks of Intel Corporation or its subsidiaries in the U.S. and/or other countries NVMe is a registered or unregistered trademark of NVM Express, Inc. in the United States and other countries. PCIe is a registered trademark of PCI-SIG.
VMware, VMware ESXi, VMware vMotion, VMware vSAN, VMware vSAN Express Storage Architecture and VMware vCenter are registered trademarks or trademarks of VMware Inc. in the United States and/or various jurisdictions.
All other company names, product names and service names may be trademarks or registered trademarks of their respective companies.
Disclaimers
© 2023 Dell, Inc. All rights reserved. Information in this tech note, including product specifications, tested content, and assessments are current and believed to be accurate as of the date that the document was published and subject to change without prior notice. Technical and application information contained here is subject to the most recent applicable product specifications.