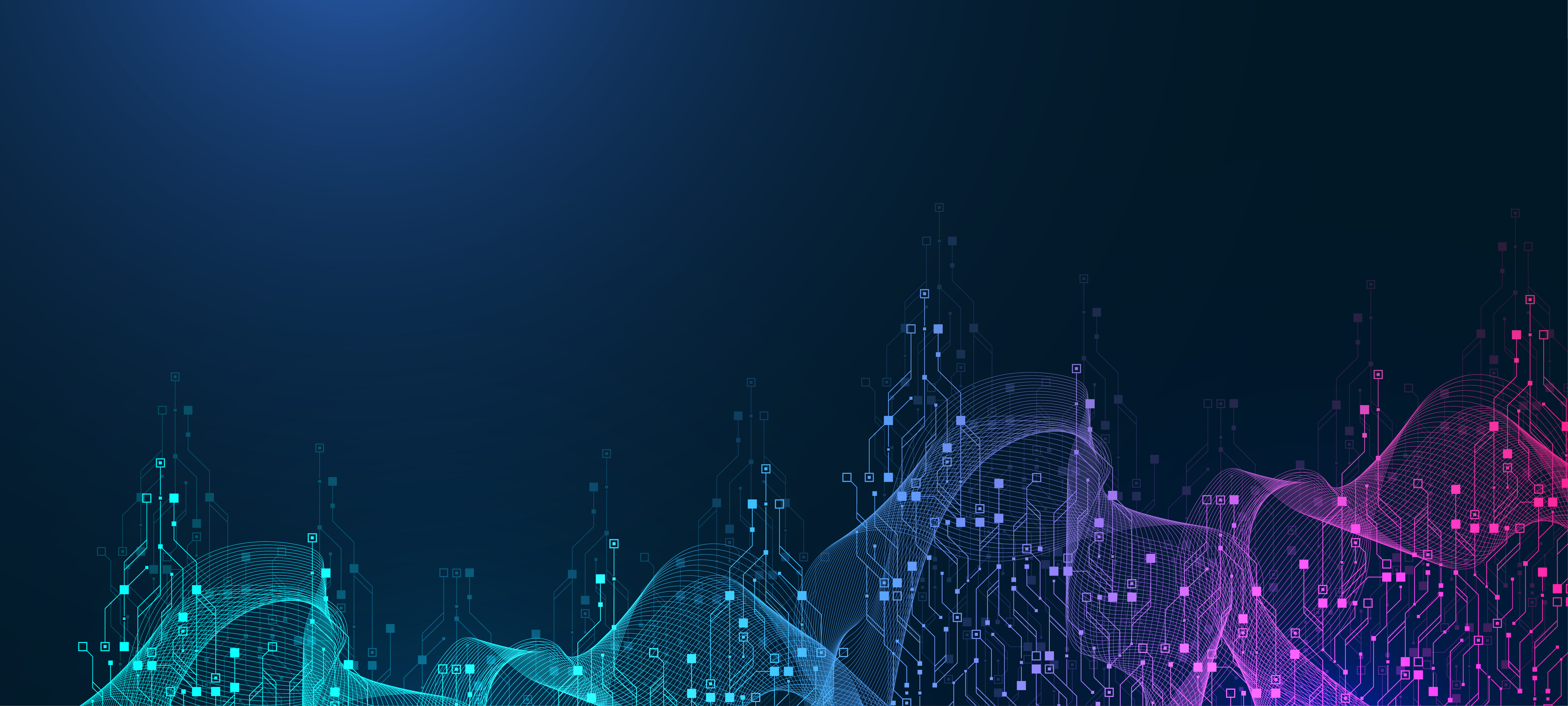
NVIDIA A100 GPU Overview
Download PDFMon, 16 Jan 2023 13:44:22 -0000
|Read Time: 0 minutes
Summary
The A100 is the next-gen NVIDIA GPU that focuses on accelerating Training, HPC and Inference workloads. The performance gains over the V100, along with various new features, show that this new GPU model has much to offer for server data centers.
This DfD will discuss the general improvements to the A100 GPU with the intention of educating customers on how they can best utilize the technology to accelerate their needs and goals.
PowerEdge Support and Benchmark Performance
The A100 will be most impactful on PCIe Gen4 compatible PowerEdge servers, such as the PowerEdge R7525, which currently supports 2 A100s and will support up to 3 A100s within the first half of 2021. PowerEdge support for the A100
GPU will roll out on different Dell EMC next-gen server platforms over the course of H1 CY21.
Figure 1 – PowerEdge R7525
Benchmarking data comparing performance on various workloads for the A100 and V100 are shown below:
Inference
Figure 2 displays the performance improvement of the A100 over the V100 for two different inference benchmarks – BERT and ResNet-50. The A100 performed 2.5x faster than the V100 on the BERT inference benchmark, and 5x faster on the RN50 inference benchmark. This will translate to significant time reductions spent on inferring trained neural networks to classify and identify known patterns and objects.

Figure 2 – Inference comparison between A100 and V100 for BERT and RN50 benchmarks
HPC
Figure 3 displays the performance improvement of the A100 over the V100 for four different HPC benchmarks. The A100 performed between 1.4x – 1.9x faster than the V100 for these benchmarks. Users looking to process data and perform complex HPC calculations will benefit from reduced completion times when using the A100 GPU.
Figure 3 – HPC comparison between A100 and V100 for GROMACS, NAMD, LAAMP and RTM benchmarks
Training
Figure 4 displays the performance improvement of the A100 over the V100 for two different training benchmarks – BERT Training TF32 and BERT Training FP16. The A100 performed 5x faster than the V100 on the BERT TF32 benchmark, and 2.5x faster on the BERT FP16 benchmark. Users looking to swiftly train their neural networks will greatly benefit from the A100 GPUs improved specs, as well as new features (such as TF32), which are further discussed below.
Figure 4 – Training comparison for BERT TF32 and FP16 benchmarks
A100 Specifications
At the heart of NVIDIA’s A100 GPU is the NVIDIA Ampere architecture, which introduces double-precision tensor cores allowing for more than 2x the throughput of the V100 – a significant reduction in simulation run times. The double-precision FP64 performance is 9.7 TFLOPS, and with tensor cores this doubles to 19.5 TFLOPS. The single-precision FP32 performance is 19.5 TFLOPS and with the new Tensor Float (TF) precision this number significantly increases to 156 TFLOPS; ~20x higher than the previous generation V100. TF32 works as a hybrid of FP16 and FP32 math models that uses the same 10-bit precision mantissa as FP16, and 8-bit exponent of FP32, allowing for speedup increases on specific benchmarks.
Furthermore, the A100 supports a massive 40GB of high-bandwidth memory (HBM2) that enables up to 1.6TB/s memory bandwidth. This is 1.7x higher memory bandwidth over the previous generation V100 (see Figure 5).
Figure 5 – A100 GPU specs
New Features
In addition to the product specification improvements noted above, the NVIDIA A100 introduces 3 key new features that will further accelerate High-Performance Computing (HPC), Training and Artificial Intelligence (AI) Inference workloads:
- 3rd Generation NVIDIA NVLink™ – The new generation of NVLink™ has 2x GPU-to-GPU throughput over the previous generation V100.
- Multi-Instance GPU (MIG) – This feature enables a single A100 GPU to be partitioned into as many as seven separate GPUs, which benefits cloud users looking to utilize their GPUs for AI inference and data analytics workloads
- Structural Sparsity – This feature supports sparse matrix operations in tensor cores and increases the throughput of tensor core operations by 2x (see Figure 6)
Figure 6 – The A100 introduces sparse matrices to accelerate AI inference tasks
User Implementation
It is important to know how the A100 can accelerate varying HPC, Training and Inference workloads:
HPC Workloads – Scientific computing simulations are typically so complex that FP64 double-precision models are required to translate the mathematics into accurate numeric models. At nearly 20 TFLOPs of double-precision performance, simulation run times are reduced by half with A100 double-precision tensor cores, allowing for 2x the normal FP64 output.
Training Workloads – Learning applications, such as recognition and training, typically require FP32 single-precision models to extract high level features from raw input. This means that the Tensor Float (TF32) computational model is an excellent alternative to FP32 for these types of applications. Running TF32 will grant up to 20x greater performance than the V100, allowing for significant train time reductions. Applications that need higher performance offered by a single server can do so by leveraging efficient scale- out techniques using low latency and high-bandwidth networking supported on the R7525. Additionally, specific training applications will also benefit from an additional 2x in performance with the new sparsity feature enabled.
Inference Workloads – Inference workloads will greatly benefit from the full range of precision models available, including FP32, FP16, INT8 and INT4. The Multi-Instance GPU (MIG) feature allows multiple networks to operate simultaneously on a single GPU so server users can have optimal utilization of compute resources. Structural sparsity support is also ideal for inference and data analytics applications, delivering up to 2x more performance on top of A100’s other inference performance gains.
Conclusion
The NVIDIA A100 GPU offers performance improvements and new feature sets that were designed to accelerate HPC, Training and AI Inference workloads. A server configured with A100 GPUs will be enabled to utilize these capabilities working in concert with other system components to yield best performance.
Related Documents
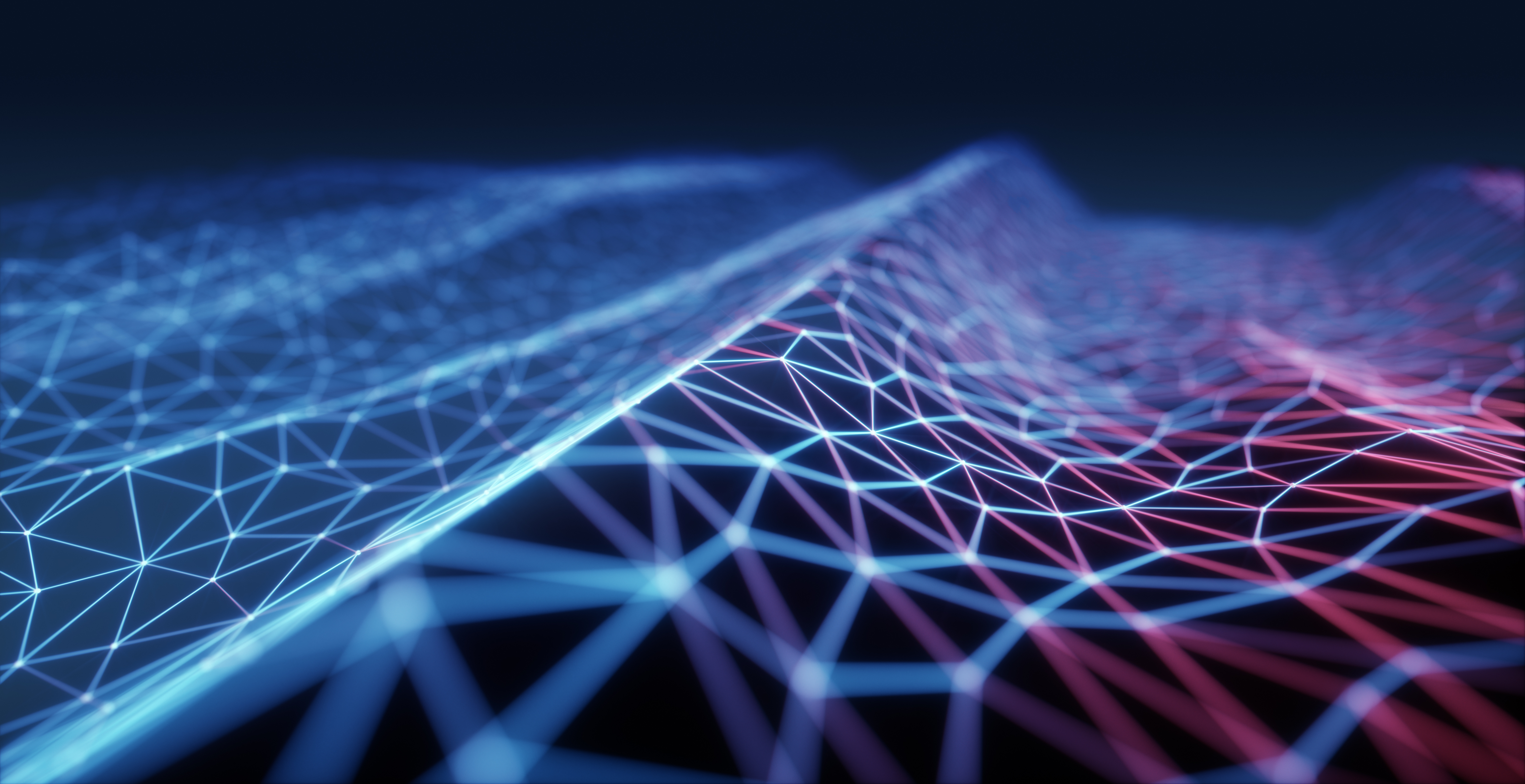
Efficient Machine Learning Inference on Dell EMC PowerEdge R7525 and R7515 Servers using NVIDIA GPUs
Mon, 16 Jan 2023 13:44:24 -0000
|Read Time: 0 minutes
Summary
Dell EMC™ participated in the MLPerf™ Consortium v0.7 result submissions for machine learning. This DfD presents results for two AMD PowerEdge™ server platforms - the R7515 and R7525. The results show that Dell EMC with AMD processor-based servers when paired with various NVIDIA GPUs offer industry-leading inference performance capability and flexibility required to match the compute requirements for AI workloads.
MLPerf Inference Benchmarks
The MLPerf (https://mlperf.org) Inference is a benchmark suite for measuring how fast Machine Learning (ML) and Deep Learning (DL) systems can process inputs and produce results using a trained model. The benchmarks belong to a very diversified set of ML use cases that are popular in the industry and provide a need for competitive hardware to perform ML-specific tasks. Hence, good performance under these benchmarks signifies a hardware setup that is well optimized for real world ML inferencing use cases. The second iteration of the suite (v0.7) has evolved to represent relevant industry use cases in the datacenter and edge. Users can compare overall system performance in AI use cases of natural language processing, medical imaging, recommendation systems and speech recognition as well as different use cases in computer vision.
MLPerf Inference v0.7
The MLPerf inference benchmark measures how fast a system can perform ML inference using a trained model with new data in a variety of deployment scenarios, see below Table 1 with the list of seven mature models included in the official v0.7 release:
Model | Reference Application | Dataset |
resnet50-v1.5 | vision / classification and detection | ImageNet (224x224) |
ssd-mobilenet 300x300 | vision / classification and detection | COCO (300x300) |
ssd-resnet34 1200x1200 | vision / classification and detection | COCO (1200x1200) |
bert | language | squad-1.1 |
dlrm | recommendation | Criteo Terabyte |
3d-unet | vision/medical imaging | BraTS 2019 |
rnnt | speech recognition | OpenSLR LibriSpeech Corpus |
The above models serve in a variety of critical inference applications or use cases known as “scenarios”. Each scenario requires different metrics, demonstrating production environment performance in real practice. MLPerf Inference consists of four evaluation scenarios: single-stream, multi-stream, server, and offline. See Table 2 below:
Scenario | Sample Use Case | Metrics |
SingleStream | Cell phone augmented reality | Latency in milliseconds |
MultiStream | Multiple camera driving assistance | Number of streams |
Server | Translation site | QPS |
Offline | Photo sorting | Inputs/second |
Executing Inference Workloads on Dell EMC PowerEdge
The PowerEdge™ R7515 and R7525 coupled with NVIDIA GPus were chosen for inference performance benchmarking because they support the precisions and capabilities required for demanding nference workloads.
Dell EMC PowerEdge™ R7515
The Dell EMC PowerEdge R7515 is a 2U, AMD-powered server that supports a single 2nd generation AMD EPYC (ROME) processor with up to 64 cores in a single socket. With 8x memory channels, it also features 16x memory module slots for a potential of 2TB using 128GB memory modules in all 16 slots. Also supported are 3-Dimensional Stack DIMMs, or 3-DS DIMMs.
SATA, SAS and NVMe drives are supported on this chassis. There are some storage options to choose from depending on the workload. Chassis configurations include:
- 8 x 3.5-inch hot plug SATA/SAS drives (HDD)
- 12 x 3.5-inch hot plug SATA/SAS drives (HDD)
- 24 x 2.5-inch hot plug SATA/SAS/NVMe drives
The R7515 is a general-purpose platform capable of handling demanding workloads and applications, such as data warehouses, ecommerce, databases, and high-performance computing (HPC). Also, the server provides extraordinary storage capacity options, making it well-suited for data-intensive applications without sacrificing I/O performance. The R7515 benchmark configuration used in testing can be seen in Table 3.
Table 3 – R7515 benchmarking configuration
Dell EMC PowerEdge™ R7525
The The Dell EMC PowerEdge R7525 is a 2-socket, 2U rack-based server that is designed to run complex workloads using highly scalable memory, I/O capacity, and network options. The system is based on the 2nd Gen AMD EPYC processor (up to 64 cores), has up to 32 DIMMs, PCI Express (PCIe) 4.0-enabled expansion slots, and supports up to three double wide 300W or six single wide 75W accelerators.
SATA, SAS and NVMe drives are supported on this chassis. There are some storage options to choose from depending on the workload. Storage configurations include:
- Front Bays
- Up to 24 x 2.5” NVMe
- Up to 16 x 2.5” SAS/SATA (SSD/HDD) and NVMe
- Up to 12 x 3.5” SAS/SATA (HDD)
- Up to 2 x 2.5” SAS/SATA/NVMe (HDD/SSD)
- Rear Bays
- Up to 2 x 2.5” SAS/SATA/NVMe (HDD/SSD)
Table 4 – R7525 benchmarking configuration
The R7525 is a highly adaptable and powerful platform capable of handling a variety of demanding workloads while also providing flexibility. The R7525 benchmark configuration used in testing can be seen in Table 4.
NVIDIA Technologies Used for Efficient Inference
NVIDIA® Tesla T4
The NVIDIA Tesla T4, based on NVIDIA’s Turing™ architecture is one of the most widely used AI inference accelerators. The Tesla T4 features NVIDIA Turing Tensor cores which enables it to accelerate all types of neural networks for images, speech, translation, and recommender systems, to name a few. Tesla T4 is supported by a wide variety of precisions and accelerates all major DL & ML frameworks, including TensorFlow, PyTorch, MXNet, Chainer, and Caffe2.
For more details on NVIDIA Tesla T4, please refer to https://www.nvidia.com/en-us/data-center/tesla-t4/
NVIDIA® Quadro RTX8000
NVIDIA® Quadro® RTX™ 8000, powered by the NVIDIA Turing™ architecture and the NVIDIA RTX platform, combines unparalleled performance and memory capacity to deliver the world’s most powerful graphics card solution for professional workflows. With 48 GB of GDDR6 memory, the NVIDIA Quadro RTX 8000 is designed to work with memory intensive workloads that create complex models, build massive architectural datasets and visualize immense data science workloads.
For more details on NVIDIA® Quadro® RTX™ 8000, please refer to https://www.nvidia.com/en-us/design- visualization/quadro/rtx-8000/
NVIDIA® A100-PCIE
The NVIDIA A100 Tensor Core GPU is the flagship product of the NVIDIA data center platform for deep learning, HPC, and data analytics. The platform accelerates over 700 HPC applications and every major deep learning framework. It’s available everywhere, from desktops to servers to cloud services, delivering both dramatic performance gains and cost-saving opportunities.
For more details, please refer to https://www.nvidia.com/en-us/data-center/a100/
NVIDIA Inference Software Stack for GPUs
At its core, NVIDIA TensorRTTM is a C++ library designed to optimize deep learning inference performance on systems which contains NVIDIA GPUs, and supports models that are trained in most of the major deep learning frameworks including, but not limited to, TensorFlow, Caffe, PyTorch, MXNet. After the neural network is trained, TensorRT enables the network to be compressed, optimized and deployed as a runtime without the overhead of a framework. It supports FP32, FP16 and INT8 precisions. To optimize the model, TensorRT builds an inference engine out of the trained model by analyzing the layers of the model and eliminating layers whose output is not used, or combining operations to perform faster calculations. The result of all these optimizations is improved latency, throughput and efficiency. TensorRT is available on NVIDIA NGC.
MLPerf v0.7 Performance Results and Key Takeaways
Figures 1 and 2 below show the inference capabilities of the PowerEdge R7515 and PowerEdge R7525 configured with different NVIDIA GPUs. Each bar graph indicates the relative performance of inference operations completed meeting certain latency constraints. Therefore, the higher the bar graph is, the higher the inference capability of the platform. Details on the different scenarios used in MLPerf inference tests (server and offline) are available at the MLPerf website. Offline scenario represents use cases where inference is done as a batch job (using AI for photo sorting), while server scenario represents an interactive inference operation (translation app). The relative performance of the different servers are plotted below to show the inference capabilities and flexibility that can be achieved using these platforms:
Offline Performance
Figure 1 – Offline scenario relative performance for five different benchmarks and four different server configs, using the R7515 (4 xT4) as a baseline
Server Performance
Figure 2 – Server scenario relative performance for five different benchmarks and four different server configs, using the R7515 (4 xT4) as a baseline
The R7515 and R7525 offers configuration flexibility to address inference performance and datacenter requirements around power and costs. Inference applications can be deployed on AMD single socket system without compromising accelerator support, storage and I/O capacities or on double socket systems with configurations that support higher capabilities. Both platforms support PCIe Gen4 links for latest GPU offerings like the A100 and also upcoming Radeon Instinct MI100 GPUs from AMD that are PCIe Gen 4 capable.
The Dell PowerEdge platforms offer a variety of PCIe riser options that enable support for multiple low- profile (up to 8 T4) or up to 3 full height double wide GPU accelerators (RTX or A100). Customers can choose the GPU model and number of GPUs based on the workload requirements and to fit their datacenter power and density needs. Figure 3 shows a relative compare of the GPUs used in the MLPerf study from a performance, power, price and memory point of view. The specs for the different GPUs supported on Dell platforms and server recommendations are covered in previous DfDs (link to the 2 papers)
Figure 3 – Relative comparisons between the A100, RTX800 and T4 GPUs for various metrics
Conclusion
As demonstrated by MLPerf performance, Inference workloads executed on Dell EMC PowerEdge R7515 and Dell EMC PowerEdge R7525 performed well in a wide range of benchmark scenarios. . These results can server a guide to help identify the configuration that matches your inference requirements.
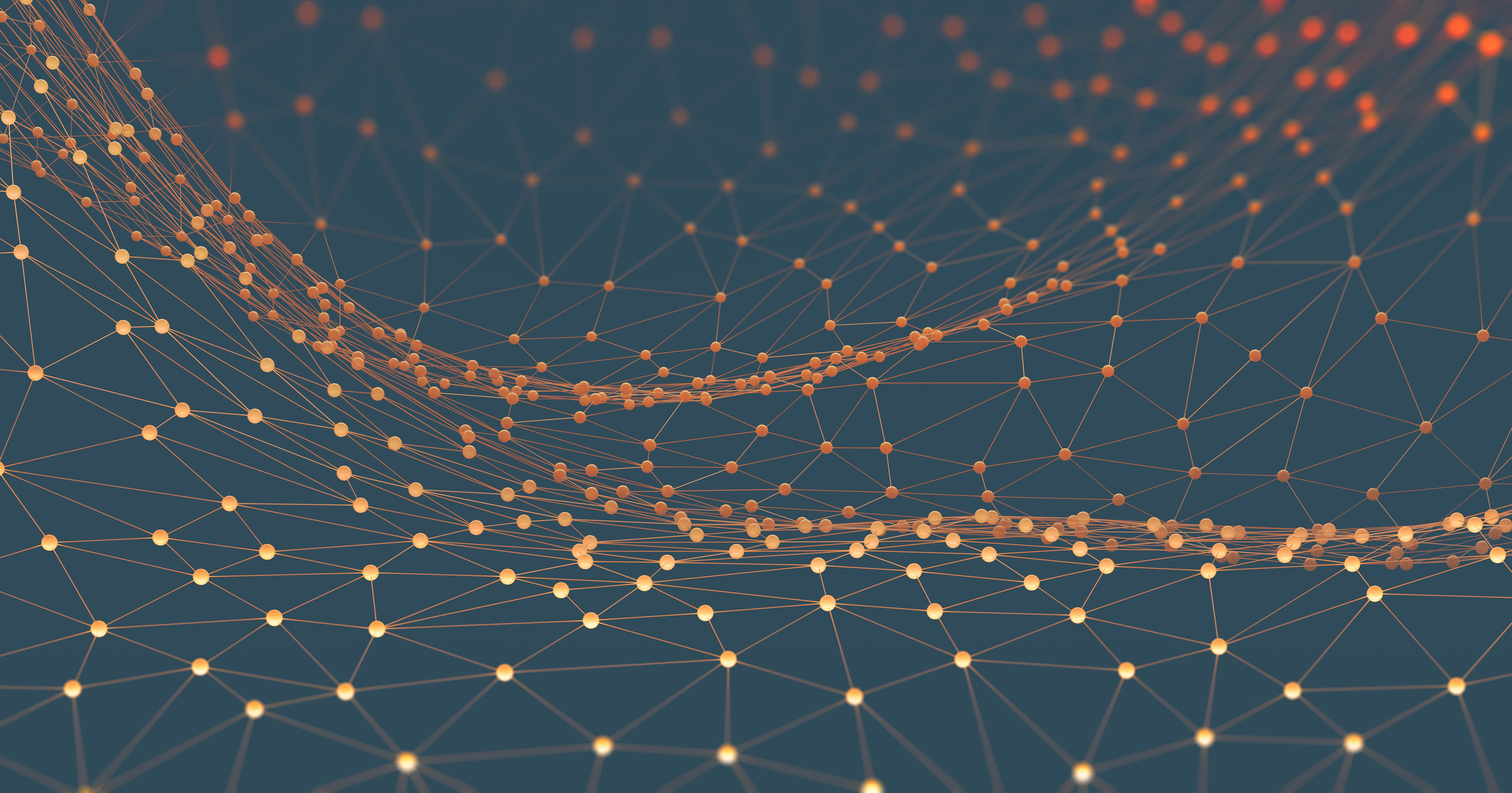
The Latest GPUs of 2022
Mon, 16 Jan 2023 13:44:30 -0000
|Read Time: 0 minutes
And How We Recommend Applying Them to Enable Breakthrough Performance
Summary
Dell Technologies offers a wide range of GPUs to address different workloads and use cases. Deciding on which GPU model and PowerEdge server to purchase, based on intended workloads, can become quite complex for customers looking to use GPU capabilities. It is important that our customers understand why specific GPUs and PowerEdge servers will work best to accelerate their intended workloads. This DfD informs customers of the latest and greatest GPU offerings in 2022, as well as which PowerEdge servers and workloads we recommend to enable breakthrough performance.
PowerEdge servers support various GPU brands and models. Each model is designed to accelerate specific demanding applications by acting as a powerful assistant to the CPU. For this reason, it is vital to understand which GPUs on PowerEdge servers will best enable breakthrough performance for varying workloads. This paper describes the latest GPUs as of Q1 2022, shown below in Figure 1, to help educate PowerEdge customers on which GPU is best suited for their specific needs.
GPU Model | Number of Cores | Peak Double Precision (FP64) | Peak Single Precision (FP32) | Peak Half Precision (FP16) | Memory Size / Bus | Memory Bandwidth | Power Consumption |
A2 | 2560 | N/A | 4.5 TFLOPS | 18 TFLOPS | 16GB GDDR6 | 200 GB/s | 40-60W |
A16 | 1280 x4 | N/A | 4.5 TFLOPS x4 | 17.9 TFLOPS x4 | 16GB GDDR6 x4 | 200 GB/s x4 | 250W |
A30 | 3804 | 5.2 TFLOPS | 10.3 TFLOPS | 165 TFLOPS | 24GB HBM2 | 933 GB/s | 165W |
A40 | 10752 | N/A | 37.4 TFLOPS | 149.7 TFLOPS | 48GB GDDR6 | 696 GB/s | 300W |
MI100 | 7680 | 11.5 TFLOPS | 23.1 TFLOPS | 184.6 TFLOPS | 32GB HBM2 | 1.2 TB/s | 300W |
A100 PCIe | 6912 | 9.7 TFLOPS | 19.5 TFLOPS | 312 TFLOPS | 80GB HBM2e | 1.93 TB/s | 300W |
A100 SXM2 | 6912 | 9.7 TFLOPS | 19.5 TFLOPS | 312 TFLOPS | 40GB HBM2 | 1.55 TB/s | 400W |
A100 SXM2 | 6912 | 9.7 TFLOPS | 19.5 TFLOPS | 312 TFLOPS | 80GB HBM2e | 2.04 TB/s | 500W |
T4 | 2560 | N/A | 8.1 TFLOPS | 65 TFLOPS | 16GB GDDR6 | 300 GB/s | 70W |
Figure 1 – Table comparing 2022 GPU specifications
NVIDIA A2
The NVIDIA A2 is an entry-level GPU intended to boost performance for AI-enabled applications. What makes this product unique is its extremely low power limit (40W-60W), compact size, and affordable price. These attributes position the A2 as the perfect “starter” GPU for users seeking performance improvements on their servers. To benefit from the performance inferencing and entry-level specifications of the A2, we suggest attaching it to mainstream PowerEdge servers, such as the R750 and R7515, which can host up to 4x and 3x A2 GPUs respectively. Edge and space/power constrained environments, such as the XR11, are also recommended, which can host up to 2x A2 GPUs. Customers can expect more PowerEdge support by H2 2022, including the PowerEdge R650, T550, R750xa, and XR12.
Supported Workloads: AI Inference, Edge, VDI, General Purpose Recommended Workloads: AI Inference, Edge, VDI Recommended PowerEdge Servers: R750, R7515, XR11
NVIDIA A16
The NVIDIA A16 is a full height, full length (FHFL) GPU card that has four GPUs connected together on a single board through a Mellanox PCIe switch. The A16 is targeted at customers requiring high-user density for VDI environments, because it shares incoming requests across four GPUs instead of just one. This will both increase the total user count and reduce queue times per request. All four GPUs have a high memory capacity (16GB DDR6 for each GPU) and memory bandwidth (200GB/s for each GPU) to support a large volume of users and varying workload types. Lastly, the NVIDIA A16 has a large number of video encoders and decoders for the best user experience in a VDI environment.
To take full advantage of the A16s capabilities, we suggest attaching it to newer PowerEdge servers that support PCIe Gen4. For Intel-based PowerEdge servers, we recommend the R750 and R750xa, which support 2x and 4x A16 GPUs, respectively. For AMD-based PowerEdge servers, we recommend the R7515 and R7525, which support 1x and 3x A16 GPUs, respectively.
Supported Workloads: VDI, Video Encoding, Video Analytics Recommended Workloads: VDI Recommended PowerEdge Servers: R750, R750xa, R7515, R7525
NVIDIA A30
The NVIDIA A30 is a mainstream GPU offering targeted at enterprise customers who seek increased performance, scalability, and flexibility in the data center. This powerhouse accelerator is a versatile GPU solution because it has excellent performance specifications for a broad spectrum of math precisions, including INT4, INT8, FP16, FP32, and FP64 models. Having the ability to run third- generation tensor core and the Multi-Instance GPU (MIG) features in unison further secures quality performance gains for big and small workloads. Lastly, it has an unconventionally low power budget of only 165W, making it a viable GPU for virtually any PowerEdge server.
Given that the A30 GPU was built to be a versatile solution for most workloads and servers, it balances both the performance and pricing to bring optimized value to our PowerEdge servers. The PowerEdge R750, R750xa, R7525, and R7515 are all great mainstream servers for enterprise customers looking to scale. For those requiring a GPU-dense server, the PowerEdge DSS8440 can hold up to 10x A30s and will be supported in Q1 2022. Lastly, the PowerEdge XR12 can support up to 2x A30s for Edge environments.
Supported Workloads: AI Inference, AI Training, HPC, Video Analytics, General Purpose Recommended Workloads: AI Inference, AI Training Recommended PowerEdge Servers: R750, R750xa, R7525, R7515, DSS8440, XR12
NVIDIA A40
The NVIDIA A40 is a FHFL GPU offering that combines advanced professional graphics with HPC and AI acceleration to boost the performance of graphics and visualization workloads, such as batch rendering, multi-display, and 3D display. By providing support for ray tracing, advanced shading, and other powerful simulation features, this GPU is a unique solution targeted at customers that require powerful virtual and physical displays. Furthermore, with 48GB of GDDR6 memory, 10,752 CUDA cores, and PCIe Gen4 support, the A40 will ensure that massive datasets and graphics workload requests are moving quickly.
To accommodate the A40s hefty power budget of 300W, we suggest customers attach it to a PowerEdge server with ample power to spare, such as the DSS8440. However, if the DSS8440 is not possible, the PowerEdge R750xa, R750, R7525, and XR12 are also compatible with the A40 GPU and will function adequately so long as they are using PSUs with adequate power output. Lastly, populating A40 GPUs within the PowerEdge T550 is also a great play for customers who want to address visually demanding workloads outside the traditional data center.
Supported Workloads: Graphics, Batch Rendering, Multi-Display, 3D Display, VR, Virtual Workstations, AI Training, AI Inference Recommended Workloads: Graphics, Bach Rendering, Multi-Display Recommended PowerEdge Servers: DSS8440, R750xa, R750, R7525, XR12, T550
NVIDIA A100
The NVIDIA A100 focuses on accelerating HPC and AI workloads. It introduces double-precision tensor cores that significantly reduce HPC simulation run times. Furthermore, the A100 includes Multi-Instance GPU (MIG) virtualization and GPU partitioning capabilities, which benefit cloud users looking to use their GPUs for AI inference and data analytics. The newly supported sparsity feature can also double the throughput of tensor core operations by exploiting the fine- grained structure in DL networks. Lastly, A100 GPUs can be inter-connected either by NVLink bridge on platforms like the R750xa and DSS8440, or by SXM4 on platforms like the PowerEdge XE8545, which increases the GPU-to- GPU bandwidth when compared to the PCIe host interface.
The PowerEdge DSS8440 is a great server for the A100, as it provides ample power and can hold the most GPUs. If not the DSS8440, we would suggest using the PowerEdge XE8545, R750xa, or R7525. Please note that only the 80GB model is supported for PCIe connections, and be sure to provide plenty of power to accommodate the A100s 300W/400W power requirements.
Supported Workloads: HPC, AI Training, AI Inference, Data Analytics, General Purpose Recommended Workloads: HPC, AI Training, AI Inference, Data Analytics Recommended PowerEdge Servers: DSS8440, XE8545, R750xa, R7525
AMD MI100
The AMD MI100 value proposition is similar to the A100 in that it will best accelerate HPC and AI workloads. At 11.5 TFLOPS, its FP64 performance is industry-leading for the acceleration of HPC workloads. Similarly, at 23.1 TFLOPs, the FP32 specifications are more than sufficient for any AI workload. Furthermore, the MI100 supports 32GB of high-bandwidth memory (HBM2) to enable a whopping 1.2TB/s of memory bandwidth. In a nutshell, this GPU is designed to tackle complex, data-intensive HPC and AI workloads for enterprise customers.
The AMD MI100 is qualified on both the Intel-based PowerEdge R750xa, which supports up to 4x MI100 GPUs, and the AMD- based PowerEdge R7525, which supports up to 3x MI100 GPUs. We highly recommend adopting a powerful PSU for either server, as the MI100 also has a massive power consumption of 300W.
Supported Workloads: HPC, AI Training, AI Inference, ML/DL Recommended Workloads: HPC, AI Training, AI Inference Recommended PowerEdge Servers: R750xa, R7525
Conclusion
The GPUs we are recommending in this list offer a wide variety of features that are designed to accelerate a diverse range of server workloads. A PowerEdge server configured with the most appropriate GPU will enable intended customer workloads to use these features in concert with other system components to yield the best performance. We hope this discussion of the latest 2022 GPUs, as well as our recommendations for Dell PowerEdge servers and workloads, will help customers choose the most appropriate GPU for their data center needs and business goals.
Learn More
Dell PowerEdge Accelerated Servers and Accelerators Dell eBook
Demystifying Deep Learning Infrastructure Choices using MLPerf Benchmark Suite HPC at Dell