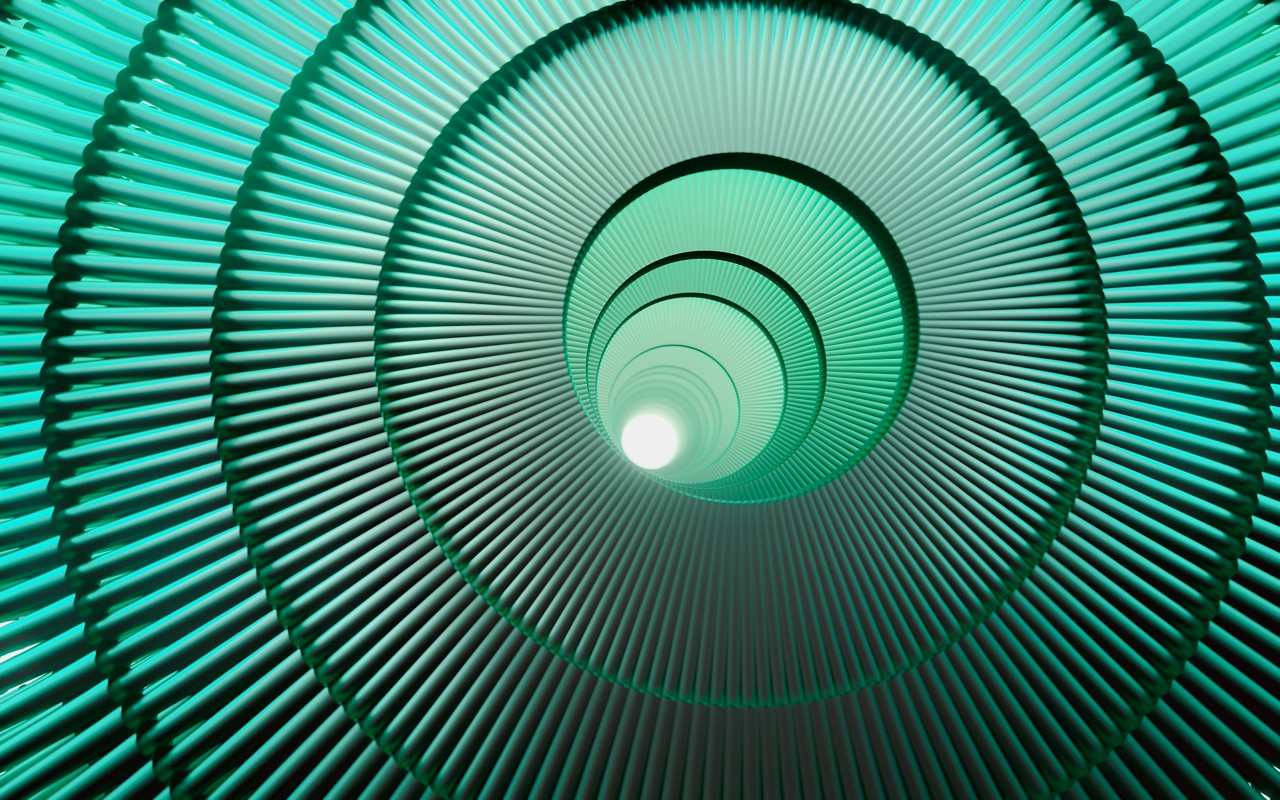
Next-Generation Dell PowerEdge XR5610 Machine Learning Performance
Download PDFFri, 03 Mar 2023 20:01:50 -0000
|Read Time: 0 minutes
Summary
Dell Technologies has recently announced the launch of next-generation Dell PowerEdge servers that deliver advanced performance and energy-efficient design.
This Direct from Development Tech Note describes the new capabilities you can expect from the next generation of PowerEdge servers. It discusses the test and results for machine learning (ML) performance of the PowerEdge XR5610 using the industry-standard MLPerf Inference v2.1 benchmarking suite. The XR5610 has target workloads in networking and communication, enterprise edge, military, and defense—all key workloads requiring AI/ML inferencing capabilities at the edge.
The 1U single-socket XR5610 is an edge-optimized short-depth rugged 1U server powered by 4th Generation Intel® Xeon® Scalable processors with the MCC SKU stack. It includes the latest generation of technologies, with slots up to 8x DDR5 and two PCIe Gen5x16 card slots, and is capable of 46 percent faster image classification (reduced latency) workload as compared to the previous-generation PowerEdge XR12.
PowerEdge XR5610—Designed for the edge
Edge computing, in essence, brings compute power close to the source of the data. As Internet of Things (IoT) endpoints and other devices generate more and more time-sensitive data, edge computing becomes increasingly important. Machine learning (ML) and artificial intelligence (AI) applications are particularly suitable for edge computing deployments. The environmental conditions for edge computing are typically vastly different than those at centralized data centers. Edge computing sites, at best, might consist of little more than a telecommunications closet with minimal or no HVAC.
Dell PowerEdge XR5610 is a rugged, short-depth (400 mm class) 1U server for the edge, designed for deployment in locations constrained by space or environmental challenges. It is well suited to operate at high temperatures ranging from –5°C to 55°C (23°F to 131°F) and designed to excel with telecom vRAN workloads, military and defense deployments, and retail AI including video monitoring, IoT device aggregation, and PoS analytics.
Figure 1. Dell PowerEdge XR5610 – 1U
The emerging technology of edge intelligence
According to a recent Forrester report, “Edge intelligence, a top 10 emerging technology in 2022, helps capture data, embed inferencing, and connect insight in a real-time network of application, device, and communication ecosystems.”
Figure 2. Forrester report excerpt, reprinted with permission
MLPerf Inference workload summary
MLPerf Inference is a multifaceted benchmark framework, measuring four different workload types and three processing scenarios. The workloads are image classification, object detection, medical imaging, speech-to-text, and natural language processing (BERT). The processing scenarios, as outlined in the following table, are single stream, multistream, and offline.
Table 1. MLPerf Inference benchmark scenarios
Scenario | Performance metric | Use case |
Single stream | 90th latency percentile | Search results. Waits until the query is made and returns the search results. Example: Google voice search |
Multistream | 99th latency percentile | Multicamera monitoring and quick decisions. Acts more like a CCTV backend system that processes multiple real-time streams and identifies suspicious behaviors. Example: Self-driving car that merges all multiple camera inputs and makes drive decisions in real time |
Offline | Measured throughput | Batch processing, also known as offline processing. Example: Google Photos service that identifies pictures, tags people, and generates an album with specific people and locations or events offline |
The MLPerf suite for inferencing includes the following benchmarks:
Table 2. MLPerf suite for inferencing benchmarks
Area | Task | Model | Dataset | QSL size | Quality |
Vision | Image classification | Resnet50-v1.5 | ImageNet (224x224) | 1024 | 99% of FP32 (76.46%) |
Vision | Object detection | Retinanet | OpenImages (800x800) | 64 | 99% of FP32 (0.3755 mAP) |
Vision | Medical image segmentation | 3D UNET | KiTS 2019 | 42 | 99% of FP32 and 99.9% of FP32 (0.86330 mean DICE score) |
Speech | Speech-to-text | RNNT | Librispeech dev-clean (samples < 15 seconds) | 2513 | 99% of FP32 (1 – WER, where WER=7.452253714852645%) |
Language | Language processing | BERT | SQuAD v1.1 (max_seq_len=384) | 10833 | 99% of FP32 (f1_score=90.874%) |
MLPerf Inference performance
The following table outlines the key specifications of the PowerEdge XR5610 that was used for the MLPerf Inference test suite.
Table 3. Dell PowerEdge XR5610 key specifications for MLPerf Inference test suite
Component | Specifications |
CPU | 4th Gen Intel Xeon Scalable processors MCC SKU |
Operating system | CentOS 8.2.2004 |
Memory | 256 GB |
GPU | NVIDIA A2 |
GPU count | 1 |
Networking | 1x ConnectX-5 IB EDR 100 Gbps |
Software stack |
|
Storage | NVMe SSD 1.8 TB |
Table 4 shows the specifications of the NVIDIA GPUs that were used in the benchmark tests.
Table 4. NVIDIA GPUs tested
GPU model | GPU memory | Maximum power consumption | Form factor | 2-way bridge | Recommended workloads |
PCIe adapter form factor | |||||
A2 | 16 GB GDDR6 | 60 W | SW, HHHL, or FHHL | Not applicable | AI inferencing, edge, VDI |
The edge server offloads the image processing to the GPU, and, just as servers have different price/performance levels to suit different requirements, so do GPUs. XR5610 supports up to 2x SW GPUs, as did the previous-generation XR11.
XR5610 was tested with the NVIDIA A2 GPU for the entire range of MLPerf workloads on the offline scenario. The following figure shows the results of the testing.
Figure 3. NVIDIA A2 GPU test results for MLPerf offline scenario
XR5610 also was tested with the NVIDIA A2 GPU for the entire range of MLPerf workloads on the single stream scenario. The following figure shows the results of that testing.
Figure 4. NVIDIA A2 GPU test results for MLPerf single stream scenario
In some tasks/workloads, the XR5610 showed improvement over previous generations, resulting from the integration of new technologies such as PCIe Gen 5.
Image classification
The PowerEdge XR5610 delivered 46 percent better image classification latency compared to the prior-generation PowerEdge server, as shown in the following figure.
Figure 5. Image classification latencies: XR5610 and prior-generation PowerEdge server
Speech to text
The Dell XR5610 delivered 15 percent better speech-to-text throughput compared to the prior-generation PowerEdge server, as shown in the following figure.
Figure 6. Speech to text latencies: XR5610 and prior-generation PowerEdge server
Conclusion
The PowerEdge XR portfolio continues to provide a streamlined approach for various edge and telecom deployment options based on different use cases. It provides a solution to the challenge of a small form factor at the edge with industry-standard rugged certifications (NEBS), providing a compact solution for scalability and for flexibility in a temperature range of –5°C to +55°C.
References
Notes:
- Based on testing conducted in Dell Cloud and Emerging Technology lab in January 2023. Results to be submitted to MLPerf in Q2, FY24.
- Unverified MLPerf v2.1 Inference. Result not verified by MLCommons Association. MLPerf name and logo are trademarks of MLCommons Association in the United States and other countries. All rights reserved. Unauthorized use strictly prohibited. See www.mlcommons.org for more information.