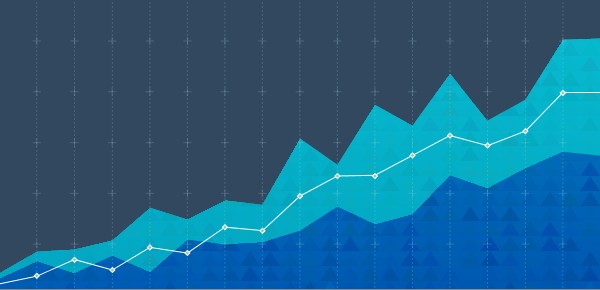
Dell Servers Excel in MLPerf™ Inference 3.0 Performance
Fri, 07 Apr 2023 10:42:23 -0000
|Read Time: 0 minutes
MLCommons has released the latest version (version 3.0) of MLPerf Inference results. Dell Technologies has been an MLCommons member and has been making submissions since the inception of the MLPerf Inference benchmark. Our latest results exhibit stellar performance from our servers and continue to shine in all areas of the benchmark including image classification, object detection, natural language processing, speech recognition, recommender system, and medical image segmentation. We encourage you to see our previous whitepaper about Inference v2.1, which introduces the MLCommons inference benchmark. AI and the most recent awareness of Generative AI, with application examples such as ChatGPT, have led to an increase in understanding about the performance objectives needed to enable customers with faster time-to-model and results. The latest results reflect the continued innovation that Dell Technologies brings to help customers achieve those performance objectives and speed up their initiatives to assess and support workloads including Generative AI in their enterprise.
What’s new with Inference 3.0?
New features for Inference 3.0 include:
- The inference benchmark rules did not make any significant changes. However, our submission has been expanded with the new generation of Dell PowerEdge servers:
- Our submission includes the new PowerEdge XE9680, XR7620, and XR5610 servers.
- Our results address new accelerators from our partners such as NVIDIA and Qualcomm.
- We submitted virtualized results with VMware running on NVIDIA AI Enterprise software and NVIDIA accelerators.
- Besides accelerator-based numbers, we submitted Intel-based CPU-only results.
Overview of results
Dell Technologies submitted 255 results across 27 different systems. The most outstanding results were generated from PowerEdge R750xa and XE9680 servers with the new H100 PCIe and SXM accelerators, respectively, as well as PowerEdge XR5610 and XR7620 servers with the L4 cards. Our overall NVIDIA-based results include the following accelerators:
- (New) Eight-way NVIDIA H100 Tensor Core GPU (SXM)
- (New) Four-way NVIDIA H100 Tensor Core GPU (PCIe)
- (New) Eight-way NVIDIA A100 Tensor Core GPU (SXM)
- Four-way NVIDIA A100 Tensor Core GPU (PCIe)
- NVIDIA A30 Tensor Core GPU
- (New) NVIDIA L4 Tensor Core GPU
- NVIDIA A2 GPU
- NVIDIA T4 GPU
We ran these accelerators on different PowerEdge XE9680, R750xa, R7525, XE8545, XR7620, XR5610 and other server configurations.
This variety of results across different servers, accelerators, and deep learning use cases allows customers to use them as datapoints to make purchasing decisions and set performance expectations.
Interesting Dell datapoints
The most interesting datapoints include:
- Among 21 submitters, Dell Technologies was one of the few companies that submitted results for all closed scenarios including data center, data center power, Edge, and Edge power.
- The PowerEdge XE9680 server procures the highest performance titles with RetinaNet Server and Offline, RNN-T Server, and BERT 99 Server benchmarks. It procures number 2 performance with Resnet Server and Offline, 3D-UNet Offline and 3D-UNet Offline 99.9, BERT 99 Offline, BERT 99.9 Server, and RNN-T Offline benchmarks.
- The PowerEdge XR5610 server procured highest system performance per watt with the NVIDIA L4 accelerator on the ResNet Single Stream, Resnet Multi Stream, RetinaNet Single Stream, RetinaNet Offline, RetinaNet Multi Stream, 3D-UNet 99, 3D-UNet 99.9 Offline, RNN-T Offline, Single Stream, BERT 99 Offline, BERT-99 Single Stream benchmarks.
- Our results included not only various systems, but also exceeded performance gains compared to the last round because of the newer generation of hardware acceleration from the newer server and accelerator.
- The Bert 99.9 benchmark was implemented with FP8 the first time. Because of the accuracy requirement, in the past, the Bert 99.9 benchmark was implemented with FP16 while all other models ran under INT8.
In the following figure, the BERT 99.9 v3.0 Offline scenario renders 843 percent more improvement compared to Inference v2.1. This result is due to the new PowerEdge XE9680 server, which is an eight–way NVIDIA H100 SXM system, compared to the previous PowerEdge XE8545 four-way NVIDIA A100 SXM. Also, the NVIDIA H100 GPU features a Transformer Engine with FP8 precision that speeds up results dramatically.
* MLPerf ID 2.1-0014 and MLPerf ID 3.0-0013
Figure 1: Performance gains from Inference v2.1 to Inference v3.0 due to the new system
Results at a glance
The following figure shows the system performance for Offline and Server scenarios. These results provide an overview as upcoming blogs will provide details about these results. High accuracy versions of the benchmark are included for DLRM and 3D-UNet as high accuracy version results are identical to the default version. For the BERT benchmark, both default and high accuracy versions are included as they are different.
Figure 2: System throughput for data center suite-submitted systems
The following figure shows the Single Stream and Multi Stream scenario latency for the ResNet, RetinaNet, 3D-UNet, RNN-T, and BERT-99 benchmarks. The lower the latency, the better the results.
Fig 3: Latency of the systems for different benchmark
Edge benchmark results include Single Stream, Multi Stream, and Offline scenarios. The following figure shows the offline scenario performance.
Figure 4: Offline Scenario system throughput for Edge suite
The preceding figures show that PowerEdge servers delivered excellent performance across various benchmarks and scenarios.
Conclusion
We have provided MLCommons-compliant submissions to the Inference 3.0 benchmark across various benchmarks and suites. These results indicate that with the newer generations of servers, such as the PowerEdge XE9680 server, and newer accelerators, such as the NVIDIA H100 GPU, customers can derive higher performance from their data center and edge deployments. Upgrading to newer hardware can yield between 304 and 843 percent improvement across all the benchmarks such as image classification, object detection, natural language processing, speech recognition, recommender systems, and medical image segmentation involved in the MLPerf inference benchmark. From our submissions for new servers such as the PowerEdge XR5610 and XR7620 servers with the NVIDIA L4 GPU, we see exceptional results. These results show that the new PowerEdge servers are an excellent edge platform choice. Furthermore, our variety of submissions to the benchmark can serve as a baseline to enable different performance expectations and cater to purchasing decisions. With these results, Dell Technologies can help fuel enterprises’ AI transformation, including Generative AI adoption, and deployment precisely and efficiently.
MLCommons Results
Related Blog Posts
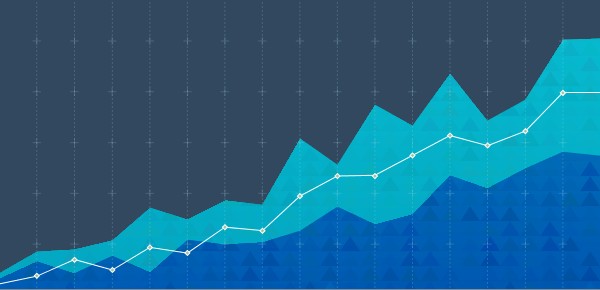
Unveiling the Power of the PowerEdge XE9680 Server on the GPT-J Model from MLPerf™ Inference
Tue, 16 Jan 2024 18:30:32 -0000
|Read Time: 0 minutes
Abstract
For the first time, the latest release of the MLPerf™ inference v3.1 benchmark includes the GPT-J model to represent large language model (LLM) performance on different systems. As a key player in the MLPerf consortium since version 0.7, Dell Technologies is back with exciting updates about the recent submission for the GPT-J model in MLPerf Inference v3.1. In this blog, we break down what these new numbers mean and present the improvements that Dell Technologies achieved with the Dell PowerEdge XE9680 server.
MLPerf inference v3.1
MLPerf inference is a standardized test for machine learning (ML) systems, allowing users to compare performance across different types of computer hardware. The test helps determine how well models, such as GPT-J, perform on various machines. Previous blogs provide a detailed MLPerf inference introduction. For in-depth details, see Introduction to MLPerf inference v1.0 Performance with Dell Servers. For step-by-step instructions for running the benchmark, see Running the MLPerf inference v1.0 Benchmark on Dell Systems. Inference version v3.1 is the seventh inference submission in which Dell Technologies has participated. The submission shows the latest system performance for different deep learning (DL) tasks and models.
Dell PowerEdge XE9680 server
The PowerEdge XE9680 server is Dell’s latest two-socket, 6U air-cooled rack server that is designed for training and inference for the most demanding ML and DL large models.
Figure 1. Dell PowerEdge XE9680 server
Key system features include:
- Two 4th Gen Intel Xeon Scalable Processors
- Up to 32 DDR5 DIMM slots
- Eight NVIDIA HGX H100 SXM 80 GB GPUs
- Up to 10 PCIe Gen5 slots to support the latest Gen5 PCIe devices and networking, enabling flexible networking design
- Up to eight U.2 SAS4/SATA SSDs (with fPERC12)/ NVMe drives (PSB direct) or up to 16 E3.S NVMe drives (PSB direct)
- A design to train and inference the most demanding ML and DL large models and run compute-intensive HPC workloads
The following figure shows a single NVIDIA H100 SXM GPU:
Figure 2. NVIDIA H100 SXM GPU
GPT-J model for inference
Language models take tokens as input and predict the probability of the next token or tokens. This method is widely used for essay generation, code development, language translation, summarization, and even understanding genetic sequences. The GPT-J model in MLPerf inference v3.1 has 6 B parameters and performs text summarization tasks on the CNN-DailyMail dataset. The model has 28 transformer layers, and a sequence length of 2048 tokens.
Performance updates
The official MLPerf inference v3.1 results for all Dell systems are published on https://mlcommons.org/benchmarks/inference-datacenter/. The PowerEdge XE9680 system ID is ID 3.1-0069.
After submitting the GPT-J model, we applied the latest firmware updates to the PowerEdge XE9680 server. The following figure shows that performance improved as a result:
Figure 3. Improvement of the PowerEdge XE9680 server on GPT-J Datacenter 99 and 99.9, Server and Offline scenarios [1]
In both 99 and 99.9 Server scenarios, the performance increased from 81.3 to an impressive 84.6. This 4.1 percent difference showcases the server's capability under randomly fed inquires in the MLPerf-defined latency restriction. In the Offline scenarios, the performance saw a notable 5.3 percent boost from 101.8 to 107.2. These results mean that the server is even more efficient and capable of handling batch-based LLM workloads.
Note: For PowerEdge XE9680 server configuration details, see https://github.com/mlcommons/inference_results_v3.1/blob/main/closed/Dell/systems/XE9680_H100_SXM_80GBx8_TRT.json
Conclusion
This blog focuses on the updates of the GPT-J model in the v3.1 submission, continuing the journey of Dell’s experience with MLPerf inference. We highlighted the improvements made to the PowerEdge XE9680 server, showing Dell's commitment to pushing the limits of ML benchmarks. As technology evolves, Dell Technologies remains a leader, constantly innovating and delivering standout results.
[1] Unverified MLPerf® v3.1 Inference Closed GPT-J. Result not verified by MLCommons Association.
The MLPerf name and logo are registered and unregistered trademarks of MLCommons Association in the United States and other countries. All rights reserved. Unauthorized use is strictly prohibited. See www.mlcommons.org for more information.
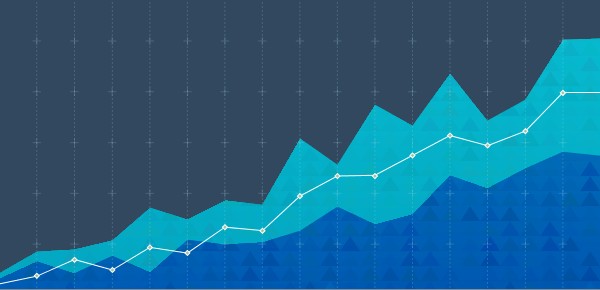
Comparison of Top Accelerators from Dell Technologies’ MLPerf™ Inference v3.0 Submission
Fri, 21 Apr 2023 21:43:39 -0000
|Read Time: 0 minutes
Abstract
Dell Technologies recently submitted results to MLPerfTM Inference v3.0 in the closed division. This blog highlights the NVIDIA H100 PCIe GPU and compares the results to the NVIDIA A100 PCIe GPU with the PCIe form factor held constant.
Introduction
MLPerf Inference v3.0 submission falls under the benchmarking pillar of the MLCommonsTM consortium with the objective to make fair comparisons across server configurations. Submissions that are made to the closed division warrant an equitable comparison of the systems.
This blog highlights the closed division submissions Dell Technologies made with the NVIDIA A100 GPU using the PCIe (peripheral component interconnect express) form factor. The PCIe form factor is an interfacing standard for connecting various high-speed components in hardware such as a computer or a server. Servers include a certain number of PCIe slots in which to insert GPUs or other additional cards. Note that there are different physical configurations for the slots to indicate the number of lanes for data to travel to and from the PCIe card. The NVIDIA H100 GPU is truly the latest and greatest GPU with NVIDIA AI Enterprise included; it is a dual-slot air cooled PCIe generation 5.0 GPU. This GPU runs at a memory bandwidth speed of over 2,000 megabits per second and up to seven Multi-Instance GPUs at 10 gigabytes each. The NVIDIA A100 80 GB GPU is a dual-slot PCIe generation 4.0 GPU that runs at a memory bandwidth speed of over 2,000 megabits per second.
NVIDIA H100 PCIe GPU and NVIDIA A100 PCIe GPU comparison
In addition to making a submission with the NVIDIA A100 GPU, Dell Technologies made a submission with the NVIDIA H100 GPU. To make a fair comparison, the systems were identical and the PCIe form factor was held constant.
Platform | Dell PowerEdge R750xa (4x A100-PCIe-80GB, TensorRT) | Dell PowerEdge R750xa (4x H100-PCIe-80GB, TensorRT) |
Round | V3.0 | |
MLPerf System ID | R750xa_A100_PCIe_80GBx4_TRT | R750xa_H100_PCIe_80GBx4_TRT |
Operating system | CentOS 8.2 | |
CPU | Intel Xeon Gold 6338 CPU @ 2.00 GHz | |
Memory | 1 TB | 1 TB |
GPU | NVIDIA A100-PCIe-80GB | NVIDIA H100-PCIe-80GB |
GPU form factor | PCIe | |
GPU memory configuration | HBM2e | |
GPU count | 4 | |
Software stack | TensorRT 8.6 CUDA 12.0 cuDNN 8.8.0 Driver 525.85.12 DALI 1.17.0 | TensorRT 8.6 CUDA 12.0 cuDNN 8.8.0 Driver 525.60.13 DALI 1.17.0 |
Table 1: Software stack of submissions made on NVIDIA A100 PCIe and NVIDIA H100 PCIe GPUs for MLPerf Inference v3.0 on the Dell PowerEdge R750xa server
In the following figure, the per card numbers are normalized over the NVIDIA A100 GPU results to show a readable comparison of the GPUs on the same system. Across object detection, medical image segmentation, and speech to text and natural language processing, the latest NVIDIA H100 GPU outperforms its predecessor in all categories. Note the outstanding performance of the Dell PowerEdge R750xa server with NVIDIA H100 GPUs with the BERT benchmark in the high accuracy mode. With the advancements in generative artificial intelligence, the Dell PowerEdge R750xa server is a versatile, reliable, and high performing platform.
Figure 1: Normalized per GPU comparison of NVIDIA A100 and NVIDIA H100 GPUs on the Dell PowerEdge R750xa server
The following figures show absolute numbers for a comparison of the NVIDIA H100 and NVIDIA A100 GPUs.
Figure 2: Per GPU comparison of NVIDIA A100 and NVIDIA H100 GPUs for RetinaNet on the PowerEdge R750xa server
Figure 3: Per GPU comparison of NVIDIA A100 and NVIDIA H100 GPUs for 3D-Unet on the PowerEdge R750xa server
Figure 4: Per GPU comparison of NVIDIA A100 and NVIDIA H100 GPUs for RNNT on the PowerEdge R750xa server
Figure 5: Per GPU comparison of NVIDIA A100 and NVIDIA H100 GPUs for BERT on the PowerEdge R750xa server
These results can be found on the MLCommons website.
Submissions made with the NVIDIA A100 PCIe GPU
In this round of submissions, Dell Technologies submitted results on the PowerEdge R750xa server packaged with four NVIDIA A100 80 GB PCIe GPUs. In previous rounds, the PowerEdge R750xa server showed outstanding performance across all the benchmarks. For a deeper dive of a previous round's submission, check out our blog from MLPerf Inference v2.0. From the previous round of MLPerf Inference v2.1 submissions, Dell Technologies submitted results on an identical system. However, across the two rounds of submissions, the main difference is the upgrades in the software stack, as described in the following table:
Platform | Dell PowerEdge R750xa (4x A100-PCIe-80GB, TensorRT) | Dell PowerEdge R750xa (4x A100-PCIe-80GB, TensorRT) |
Round | V3.0 | V2.1 |
MLPerf System ID | R750xa_A100_PCIe_80GBx4_TRT | |
Operating system | CentOS 8.2 | |
CPU | Intel Xeon Gold 6338 CPU @ 2.00 GHz | |
Memory | 512 GB | |
GPU | NVIDIA A100-PCIe-80GB | |
GPU form factor | PCIe | |
GPU memory configuration | HBM2e | |
GPU count | 4 | |
Software stack | TensorRT 8.6 CUDA 12.0 cuDNN 8.8.0 Driver 525.85.12 DALI 1.17.0 | TensorRT 8.4.2 CUDA 11.6 cuDNN 8.4.1 Driver 510.39.01 DALI 0.31.0 |
Table 2: Software stack for submissions made on the NVIDIA A100 PCIe GPU in MLPerf Inference v3.0 and v2.1
Comparison of PowerEdge R750xa NVIDIA A100 results from Inference v3.0 and v2.1
Object detection
The RetinaNet benchmark falls under the object detection category and uses the OpenImages dataset. The results from Inference v3.0 show a less than 0.05 percent difference in the Server scenario and a 21.53 percent difference in the Offline scenario. A potential reason for this result might be NVIDIA’s optimizations, as outlined in their technical blog.
Figure 6: RetinaNet Server and Offline results on the PowerEdge R750xa server from Inference v3.0 and Inference v2.1
Medical image segmentation
The 3D-Unet benchmark performs the KiTS 2019 kidney tumor segmentation task. Across the two rounds of submission, the PowerEdge R750xa server performed consistently well with a 0.3 percent difference in both the default and high accuracy modes.
Figure 7: 3D-UNet Offline results on the PowerEdge R750xa server from Inference v3.0 and v2.1
Speech to text
The Recurrent Neural Network Transducers (RNNT) model falls under the speech recognition category. This benchmark accepts raw audio samples and produces the corresponding character transcription. In the Server scenario, the results are within a 2.25 percent difference and 0.41 percent difference in the Offline scenario.
Figure 8: RNNT Server and Offline results on the Dell PowerEdge R750xa server from Inference v3.0 and v2.1
Natural language processing
Bidirectional Encoder Representation from Transformers (BERT) is a state-of-the-art language representational model for Natural Language Processing applications. This benchmark performs the SQuAD question answering task. The BERT benchmark consists of default and high accuracy modes for the Offline and Server scenarios. For the Server scenarios, the default mode results are within a 1.69 percent range and 3.12 percent range for the high accuracy mode. For the Offline scenarios, a similar behavior is noticeable in which the default mode results are within a 0.86 percent range and 3.65 percent range in the high accuracy mode.
Figure 9: BERT Server and Offline results on the PowerEdge R750xa server from Inference v3.0 and v2.1
Conclusion
Across the various rounds of submissions to the MLPerf Inference benchmark suite, the PowerEdge R750xa server has been a consistent top performer for any machine learning tasks ranging from object detection, medical image segmentation, speech to text and natural language processing. The PowerEdge R750xa server continues to be an excellent server choice for machine learning inference workloads. Customers can take advantage of the diverse results submitted on the Dell PowerEdge R750xa server with the NVIDIA H100 GPU to make an informed decision for their specific solution needs.